Love, Death, and Pitching Robots: Designing a Hurler Archetype to Survive the Latest Wave of Baseball Tech
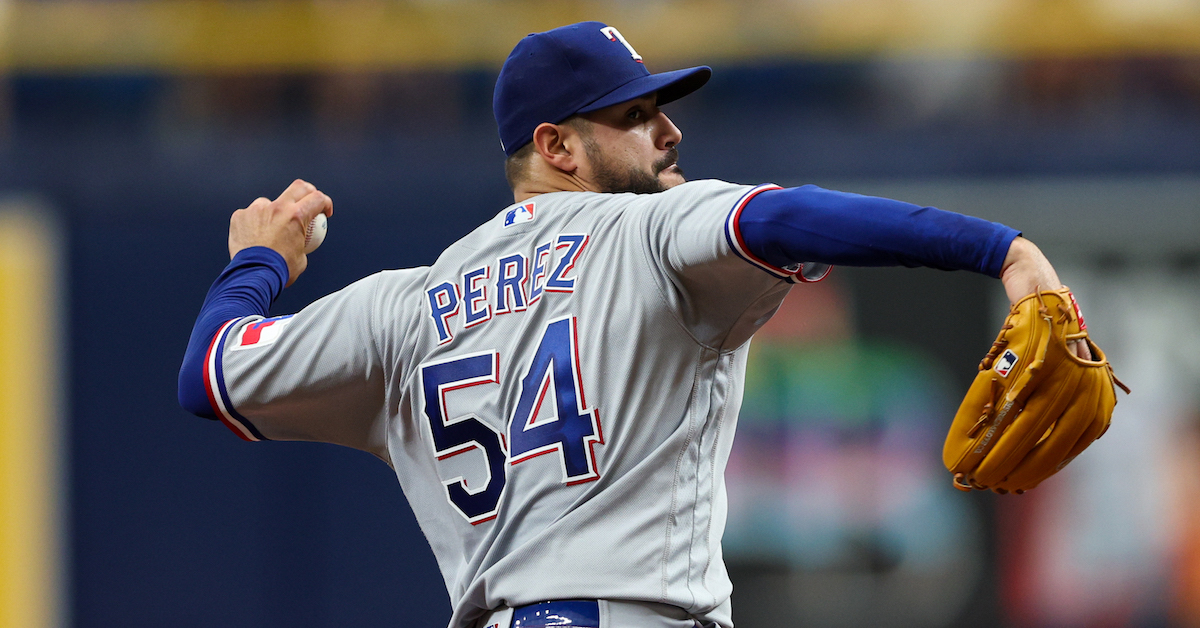
The context behind the phrase “pitch tipping” has grown richer every year. Sure, the basic principle still holds: a pitcher is “tipping” when they’re providing some indication of their upcoming offerings. It’s just that opponents can glean such “tips” through a continuously expanding network of avenues. Previously, the only [clears throat] legal way to do so was when a second-base runner or base coach picked up on a catcher’s signs, or a starting pitcher’s tendency to wind up differently for a fastball or a breaking ball. Then, with the advent of PITCHf/x and later Trackman and Hawkeye, analysts and machine learning algorithms could search for tips to cue their hitters — when Pitcher A throws from a higher release point, there’s usually a fastball coming; when he shortens up his stride, there will probably be a breaking ball.
Next, the Trajekt pitching robots made it so that not only could coaches convey these cues to their hitters, but they could demonstrate how to use them to their advantage in real time. Integrating near-perfect trajectory replication with video of each pitcher’s windup, a pitcher facsimile completes their follow through at a mobile slot — adjustable in three dimensions for different release points and extensions — from which a batting practice baseball is launched. Still, pitchers can make in-game adjustments and at least avoid falling prey to the Trajekt machine for one start at a time, and the use of PitchCom makes it harder for runners and coaches to become privy to signs in-game. Maybe all of that can at least spare the pitcher an inning?
Now, I’m not so sure. Last week, Sports Illustrated’s Tom Verducci described team executives and coaches who are spending more time combatting their hurlers’ tipping than ever before. That’s because of markerless motion capture systems installed in as many as 15 big league ballparks. There are supposedly safeguards against using these KinaTrax systems for sign stealing, safeguards that dovetail with PitchCom’s effects, but the cameras go far beyond their intended purpose of preventing injury and sharpening up mechanics. Verducci describes an example, relayed to him from a front office executive: pitch grip influences which forearm muscles activate and how much they activate, even while the ball is still in the pitcher’s glove; once analysts or machine learning algorithms match each flexion pattern to a particular pitch type, that information goes straight to the dugout, and then to the hitters.
For the time being, all of this analysis is legal, whether it’s conducted post- or in-game. There’s a chance that it won’t be for long, or that it will at least be capped in some meaningful way pretty soon. But in the meantime, this could be a serious issue for hurlers; Zach Crizer and Hannah Keyser reported last year that eight teams would have access to Trajekt robots by the end of the 2022 season, and that number has surely only grown since. No machine integrates video quite like Trajekt, but 20 teams (and Driveline) are using a Spinball iPitch, which similarly tries to replicate spin rates and velocity. There are multiple other similar products out there, including Sports Attack’s Hack Attack; I wouldn’t be surprised to learn that 90% of teams have access to one intelligent pitching machine or another, even if some of them are still working out how to best integrate them into their gameday preparation plans.
So if you’re a pitcher, or a team searching for good pitching, the question becomes: how the heck can you counteract this? One possibility would be to focus more on wrist action; that way, you could have a pitch that fools the algorithms by activating the same forearm muscles as another due to grip similarity, but moves in an entirely different direction due to a subtle mechanical change that can’t be picked up when the pitcher is in the set position. Think of Ian Hamilton’s slambio, which I wrote about in April. The pitch runs the gamut from gyro to cutting action depending on the degree of Hamilton’s pronation. It’s possible hitters would adjust to focus on pronation as a cue, but wrist action happens so fast that I think it would take some time for batters to get there.
One complication to this idea is that right now, pitchers are being taught to lean into whatever their natural wrist action is; if they’re a supinator, they should focus on pitches like sweepers that require supination and use a splitter for their offspeed look, while pronators are typically better off avoiding the sweeper and focusing on a straight or a circle change. Contrary to what some in the old guard might believe, there doesn’t seem to be any difference in the incidence of injuries for natural pronators versus supinators. On the other hand, the lean-into-what’s-natural pitching philosophy dovetails with the fact that it’s hard to manipulate wrist action; attempting to do so can lead to two different pitches, like a curve and a slider, blending together. But I also couldn’t find anything to suggest that employing both pronation and supination leads to greater injury risk, so it could be worth a shot. Even if I’m mistaken, messing with finger pressure post-separation could be another way to alter movement while still avoiding KinaTrax detection.
One way to identify pitchers who could excel in this new world is by looking at spin axis relative to movement. We can’t intuit their exact grip on the ball, i.e., the number of fingers they’re using, with public data, so the line around which the pitched ball spins will have to do as a proxy. My thinking here is that if a pitcher spins the ball around the same axis every time, it’s less likely there will be discernible differences in their forearm flexion. Yet it’s also less likely there will be much difference in movement from pitch to pitch. So combining the best of both worlds, we should be looking for pitchers who have the lowest standard deviation in spin axis and the highest in horizontal and vertical movement. Spin axis is also influenced by wrist action and finger pressure, so we may end up finding pitchers with stiff wrists and fingers, but if they’re getting more movement than expected that way, they could at least preempt the potential wrist-action counter-revolution I mentioned above.
Before we get there, let’s start by firmly establishing the relationship between movement and spin axis. Spin axis data can be tough to work with since a one-degree difference can take on very disparate meanings depending on where you start from. For example, a one-degree increase in spin axis when you’re already at 100 degrees will get you to 101, but a one-degree increase when you’re at 360 degrees will get you all the way back to one. I’m no trigonometry whiz, but we should be able to capture all of the relevant properties of spin axis by taking the sine and cosine of our initial angle and storing those values in two separate variables. As a refresher, sine and cosine both range from -1 to 1; here are some landmark values for the two measurements:
Angle (Degrees) | 0 | 30 | 60 | 90 | 180 | 270 | 360 |
---|---|---|---|---|---|---|---|
Sin | 0 | 1/2 | √3/2 | 1 | 0 | -1 | 0 |
Cos | 1 | √3/2 | 1/2 | 0 | -1 | 0 | 1 |
Sure enough, with each point representing a single pitch (and yes, that broke our poor graphing tool), the sine of spin axis has explained 78.4% of the variation in horizontal movement (without gravity) so far this season:
And the cosine has explained 63.3% of the variation in vertical movement:
For what it’s worth, adding spin rate hardly boosts those numbers at all, to 78.41% and 64%, respectively.
Using our handy benchmarks, the first graph is telling us that horizontal movement is maximized with spin axes around 90 degrees (sines around 1). These pitches are mostly right-handed pitcher sweepers and sliders, with some lefty changeups mixed in. The opposite goes for when horizontal movement is minimized (or, arm-side run is maximized for righties); these pitches, with spin axes around 270 degrees (sines around -1), are mostly lefty sweepers and sliders, with some righty changeups mixed in. Horizontal movement is close to zero with very high or low spin axes; these are your 12-6 curves and your curveball-like sliders, with some gyros as well. Gyros don’t move much outside of gravity’s influence due to their bullet-like spin, but they also end up in this category simply because Statcast provides spin axis in only two dimensions; in order to incorporate gyro spin, you need three dimensions or a measurement of active spin. Consider Luis Castillo’s gyroball:
The spin axis seems like it’s all over the place, but really the system is just struggling to pick it up.
As for the cosine graph, vertical movement is maximized with spin axes around 180 degrees (cosines around -1). These are mostly cutters, four-seamers, and hard (and/or gyro) sliders. Drop is maximized with very high or low spin axes (cosines around 1) — our curves, curve-like sliders, and gyros again — and vertical movement is close to zero when cos is zero, at 90 or 270 — our sweepers, sliders, and running changeups again.
With that out of the way, let’s return to the task at hand — searching for pitchers without much variation in their spin axes but a lot of variation in their pitch movements. Gyroballs make this task difficult because their two-dimensional spin axes are so variable; when they’re included, the correlation between the standard deviation (SD) of spin axis cosines and the SD of vertical movement (among pitchers who’ve thrown at least 500 pitches this year) is .43 by r-squared. Without them (i.e., without pitches with 25% or less spin efficiency), that number jumps to .66. Similarly, the correlation between the SD of spin axis sines and the SD of horizontal movement is .45 with gyroballs and .80 without them. The calculations I used to get spin efficiency come from Dr. Alan Nathan.
For now, let’s eschew gyroballs. Below are the graphs for all 155 pitchers who’ve thrown at least 500 non-gyroballs this season; each point represents the entire arsenal of one of those 155 pitchers:
I picked on Martín Pérez, encircled in red, because he stood out as having a low spin axis SD and a sizable positive residual (i.e., he had more movement than expected given his spin axis variation). Pérez actually throws a wide variety of pitches, but he relies primarily on a cutter, sinker, and changeup — those three offerings have comprised 94% of his pitches this season. Pérez’s sinker and changeup spin around nearly identical axes, and his cutter is just a bit closer to a pure backspinner. But all of these pitches have significantly different grips, especially the changeup, which he seems to be throwing with all of his fingers on the ball:
This is probably the same circle change he’s thrown since 2021, when he emphasized to my colleague David Laurila how much he works to have the same arm action on the pitch as his fastball.
For the cosine graph, I picked on Kevin Gausman, encircled in red:
Part of the reason for his position here is because his slider, which he’s thrown 9.5% of the time this season, is mostly left out due to its low spin efficiency. The other factor is that he throws his splitter 38.6% of the time, and its low spin rate subjects it to the whims of gravity but also mercurial wind and other weather-related influences like a knuckleball — it’s no wonder the pitch has such variable movement. His main offering, a four-seamer thrown 51.5% of the time, is spun about a similar axis to the split — hence the low standard deviation of spin axis cosines — just with considerably more backspin.
Like Pérez, Gausman utilizes different grips for his main offerings and is thus still vulnerable to the KinaTrax cameras. It could explain why he has the occasional blowup, but this isn’t what I was aiming to unearth with my study. Perhaps I should adjust the scale and zoom in, moving from analyzing entire arsenals to single pitches. This new kind of investigation wouldn’t be without its own problems — namely, once individual pitches reach a certain level of movement variability, they are more likely to diverge into two separate pitch classifications — but it would represent another step towards controlling for grip and could allow for the inclusion of gyroballs. Until then, may the KinaTrax gods have mercy on us all.
Stats are as of end of day Tuesday, June 13.
Alex is a FanGraphs contributor. His work has also appeared at Pinstripe Alley, Pitcher List, and Sports Info Solutions. He is especially interested in how and why players make decisions, something he struggles with in daily life. You can find him on Twitter @Mind_OverBatter.
Robots 3 Humans 0.