Swing-Mirroring 2, Eclectic Boogaloo
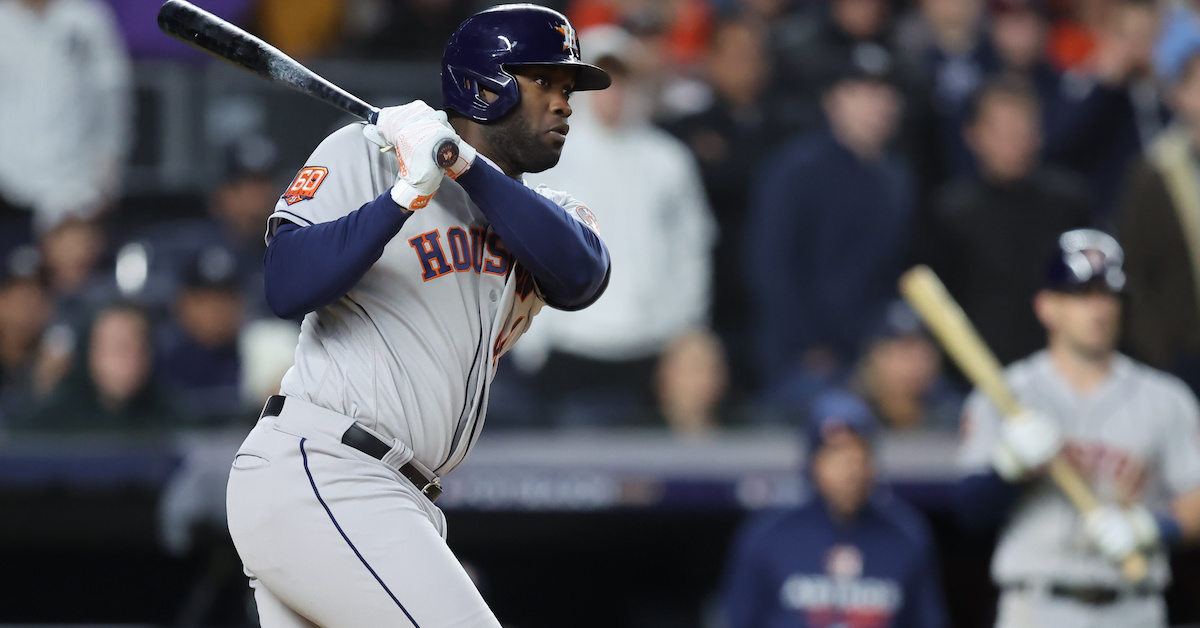
In my last article on swing-mirroring, I detailed how, on average, one hitter’s result impacts the first-pitch swing decisions of the next hitter. I was inspired by Asch Conformity, or social influence, something I’ve experienced in my own life whenever I’ve been the last among family and friends to tune into a TV show or movie series. Usually, I cave and watch because I either want to be able to join cultural conversations and/or I convince myself that if everyone else likes a piece of media, so will I. These reasons typify the two general types of social influence: Normative, or when you are enticed to conform for the sake of fitting in; and informational, or when you conform because you think doing so is the right course of action (i.e., maybe I’ll actually enjoy the TV show).
Going back to baseball, each offensive result didn’t fall neatly into either category of social influence (nothing in life truly does). Additionally, for some results like double plays, other psychological factors such as reference dependence played a part. So I instead went very general and ended up categorizing outcomes based on whether they tended to increase, decrease, or have no consistent impact on the subsequent hitters’ first-pitch swing rate (FPS%).
This process served as a lesson in how difficult it can be to disentangle individual psychological drivers of behavior from the broader workings of the environment and the mind, especially when using observational data. But at the same time, I also noticed that the general trends varied based on the first-pitch swinger in question. This opened up another avenue to explore: Examining the patterns of individual differences in swing-mirroring could get me closer to isolating the effect of social influence.
The initial groupings I came up with were as follows: strikeouts and hits tended to increase FPS%; field outs (especially double plays) tended to lower it; and bunts, errors (lumped in with all-safe fielder’s choices), walks, and hit by pitches had inconsistent effects on FPS%. Not all of these outcomes occur frequently enough in one season to measure on an individual-player basis, so I did my best to combine similar ones without sacrificing nuance. I combined all kinds of strikeouts (called, swinging, and looking), and found that 167 players batted after at least 50 strikeouts in both 2021 and ’22; I combined singles and doubles and found that 192 players hit after at least 50 singles and/or doubles in both 2021 and ’22; and I looked at all field outs and found that 289 players strode to the plate after at least 50 field outs in both 2021 and ’22. Other attempts at categorizing outcomes, including walks plus hit-by-pitches, didn’t yield nearly as many players year over year.
Before getting too into the weeds, I wanted to make sure that these categories still significantly impacted FPS% with some additional controls. Besides outs when up and with the lead runner on, both of which I used in my last article and represent the influence of “urgency” in determining first-pitch swings, I also included inning, score differential, and whether or not the first pitch in question landed within the Statcast strike zone. Because there was no difference in FPS% hitting with one or two outs, but there was between hitting with any number of outs versus hitting with zero, I codified the outs variable as “1” if the number of outs when up was nonzero and as “0” otherwise.
For my assessment, I performed a logistic regression, which can be used to determine how each of a set of predictors impacts the odds of an event (typically with a binary outcome) holding the other predictors constant. The event in this scenario is a first-pitch swing (the other possible outcome is a take), and the data comprises all first pitches for a given season. All of the controls were statistically significant, with the strike variable having the largest effect: going from a ball to a strike, all else constant, increased the odds of a swing nearly fivefold (500%) for 2021 and fourfold for ’22. This is good news for hitters: they aren’t completely beholden to the potentially subconscious and insidious influences of urgency and social influence. Rather, the single most determinative factor is whether the pitch is a strike; writ large, hitters will swing at good first pitches to hit more often than bad ones. The next largest impact among the controls was the lead runner variable: when the lead runner moved up one base, the odds of a swing increased by 17.2% in 2021 and 14.8% in ’22.
Meanwhile, when it came to the main variables of interest, the single/double and strikeout categories both performed as expected. Both were statistically significant; following a single or double increased the odds of a swing by 17% in 2021 and 15.3% in ’22, and following a strikeout increased the odds by 20.5% in 2021 and 19.3% in ’22. Field outs did not significantly alter the odds of a swing, however; they decreased them by a mere 0.004% in both years. For what it’s worth, I included walks plus hit-by-pitches in one iteration of the regression, and they did significantly increase the odds of a swing, by 6.9% in 2021 and 5.2% in ’22. However, this is likely because bases-empty situations, which rarely follow walks and hit-by-pitches, produce the lowest FPS%. Walks and HBPs only appear to increase FPS% because they exclude bases-empty situations.
Given the insignificance of field outs and the issues (sample size, confounding factors) with walks plus hit-by-pitches, I decided to focus my analysis on the single/double and post-strikeout groups. With the goal of isolating the effects of social influence, my main empirical question was whether swing-mirroring susceptibility was sticky; in other words, if a player’s FPS% increased by more than average following a single/double or a strikeout in 2021, would it do so in ’22 as well?
Before digging into this question, I had to do some more data work. For each hitter in the post-strikeout group of 167, I compiled their FPS% following every outcome besides a strikeout for both 2021 and ’22. I then looked at their FPS% following strikeouts for both years. To answer my question, I needed the difference between these two averages for each hitter each year; who swung way more than their usual rate following a strikeout, and who swung way less? Those who swing far more may be especially susceptible to social influence given the tendency on average to swing more following a teammate’s strikeout. The differences came out messy, so I normalized them, taking the Z-score of every hitter’s difference.
Heads up for a statistical primer; feel free to skip this paragraph if you’re already familiar with Z-scores or are more interested in my bottom line. A Z-score in this case is just the number of standard deviations a particular hitter’s difference is away from the mean difference. The mean difference among the group for 2021 (FPS% post-non-strikeout minus FPS% post-strikeout) was -3.0%. The average standard deviation, or difference from the mean difference, was 6.5%. So a Z-score of 0 indicates an FPS rate 3% higher after strikeouts (i.e., zero standard deviations removed from the mean), a Z-score of 1 delineates an FPS rate 3.5% lower after strikeouts (hitters less susceptible to social influence than average or even anti-conformists), and a Z-score of -1 denotes an FPS rate 9.5% higher after strikeouts (hitters more susceptible to social influence than average). For each hitter in the single/double group of 192, I conducted the same procedure, taking the difference between FPS% following outcomes besides singles/doubles and FPS% following singles/doubles. The mean differences and standard deviations here were -5.0% and 6.6%, respectively, for 2021, and -3.9% and 6.5% for ’22. I normalized those as well.
Other – Ks | Other – Ks | Other – (1Bs + 2Bs) | Other – (1Bs + 2Bs) | |
---|---|---|---|---|
Year | 2021 | 2022 | 2021 | 2022 |
Mean | -3.0 | -2.3 | -5.0 | -3.9 |
SD | 6.5 | 7.1 | 6.6 | 6.5 |
Z = 0 | -3.0 | -2.3 | -5.0 | -3.9 |
Z = 1 | 3.5 | 4.8 | 1.6 | 2.6 |
Z = -1 | -9.5 | -9.4 | -11.6 | -10.4 |
Data digging complete, my first attempt at answering the stickiness question was predicting (for both the post-strikeout and singles/doubles group) the normalized differences in 2022 using the normalized differences from ’21. I regressed the 2022 values on those for ’21, as well as the controls I listed previously. Given that I was attempting to predict 2022 values, the controls were gleaned only from 2022 data.
To start, I tackled first pitches following strikeouts. I included two sets of the same 2022 control variables, one for post-strikeout first pitches and one for first pitches following other outcomes. Collinearity, or correlations among these predictors, was surprisingly limited; the differences across the two sets of control variables were sufficient enough that it warranted including both sets. This indicates that, at least when it comes to the Statcast strike variable, pitchers are likely approaching hitters differently depending on the previous outcome — perhaps an article for another day. The only control I omitted was outs when up post-strikeout, since the number of outs was always nonzero in this instance. I did include outs when up following other outcomes.
Controls constant, the normalized differences from 2021 did significantly predict those from ’22. Specifically, for every one-point increase in 2021 Z-score, it increased the following year by 0.17 on average — hardly a one-to-one correspondence, but statistically significant nonetheless. The only control that was significant was Statcast strike rate, both for first pitches following strikeouts and first pitches following other outcomes. For every 1% increase in strike rate following strikeouts, Z-score decreased by 0.07; for every 1% increase in strike rate following other outcomes, Z-score increased by 0.08. This is because first-pitch strikes encourage first-pitch swings. So when strike rate increases after a strikeout, the number on the right of the minus sign increases (post-other outcome FPS% – post-strikeout FPS%), making the normalized difference more negative; when strike rate increases after other outcomes, the number to the left of the minus sign increases, making the normalized difference more positive.
Given that so many of the controls were insignificant predictors, I used a technique called backward elimination to remove the least helpful predictors one by one until only helpful ones remained. Sometimes, insignificant predictors will soak up predictability that might otherwise be attributed to other variables. So in redistributing soaked-up predictability, backward elimination can unearth variables that might be more powerful than initially thought.
After the elimination process, the controls for inning and score differential post-strikeout remained in addition to the initial three variables, though score differential only improved the model’s predictability and was not itself statistically significant. In this reduced model, 2021 Z-score was still a significant predictor, with a one-point increase corresponding with a 0.15 increase in Z-score the following year. Additionally, for every one-inning increase, 2022 Z-score decreased by 0.34. Urgency is heightened in later innings, increasing FPS%; in later innings after a strikeout, the number on the right of the minus sign increases, making the Z-score more negative. Why did only the post-strikeout inning control stick around? My guess would be because of the absence of meaningful influence from the number of outs in the post-strikeout case. More outs and more innings both lead to more urgency, so the two controls typically encroach on each other a bit.
Returning to the singles/doubles group, 2021 Z-score was an even more powerful predictor of ’22 Z-score, with a one-point increase in ’21 corresponding with a 0.29 increase in ’22. Only Statcast strike rate following singles and/or doubles was significant otherwise; a 1% increase in strike rate led to a 0.07 decrease in 2022 Z-score (because the number on the right of the minus sign increased).
In the backward elimination model, outs when up, runners on, and inning (all post-other outcomes) remained in addition to the initial two variables, but only runners on was significant. There were almost always runners on in the post-singles/doubles situations, so that’s likely why this control wasn’t as important there. Regardless, 2021 Z-score was still significant in the reduced model, with a one-point increase corresponding with a 0.27 increase in ’22 Z-score. For every one-base increase on average in post-other outcomes situations, Z-score decreased by a whopping 2.39. Typically, the lead runner moving up causes more urgency and higher FPS rates, which would increase the number to the left of the minus sign and the overall Z-score; in this case, it decreased that number.
Advancing the lead runner should increase FPS% regardless of the previous outcome. My best guess would be that, since there is some overlap (though still not enough to cause collinearity) between average lead runner across the outcomes of interest for each hitter (potentially caused by a typical lineup spot), more runners closer to scoring in the post-other situations mean even more runners closer to scoring in the post-single/double situations. This is because, proportionally, there are typically more runners on in post-single/double situations:
Post-Single/Double? | Lead Runner on | FPS% | Proportion of All |
---|---|---|---|
No | N/A | 28.6 | 68.3 |
No | 1st | 33.2 | 14.4 |
No | 2nd | 33.0 | 9.9 |
No | 3rd | 35.3 | 7.4 |
Yes | N/A | 33.1 | 0.5 |
Yes | 1st | 33.9 | 48.7 |
Yes | 2nd | 33.9 | 34.3 |
Yes | 3rd | 39.2 | 16.5 |
Whew, that was a lot. If you glossed over the regression stuff, welcome back. I’ll be the first to admit that I tend to get lost in the intricacies of my analysis; when what you do for a living is write about ever-thinner slices of a strategic game, it’s hard not to get bogged down by the details. Regardless, my main takeaway was this: there is evidence that both individual tendencies to swing more after strikeouts and after singles/doubles are somewhat sticky year-over-year. In other words, it’s possible that what I’m getting at here is some trait-level susceptibility to social influence among baseball players, which is a pretty amazing thing to be able to extract from pitch-by-pitch data.
But I still have more questions. How do individual differences among pitchers play into all this? Are the conformists in the single/double condition the same as the conformists in the strikeout condition? Does being a conformist in either condition meaningfully impact performance yet, and if not, will it in the future? To come full circle, I know that my TV-watching is meaningfully impacted by my desire to fit in; sometimes this leads to a new favorite show (like with Ted Lasso) and other times this leads to me being freaked out (like with Attack On Titan). More nefariously, sometimes this leads to people taking advantage of me. Is this the case with hitters? Sometimes their subconscious push to swing on 0–0 rewards them with a hit; other times, it ends in a whiff. In the future, maybe pitchers will pounce on such tendencies. Stay tuned to find out.
Alex is a FanGraphs contributor. His work has also appeared at Pinstripe Alley, Pitcher List, and Sports Info Solutions. He is especially interested in how and why players make decisions, something he struggles with in daily life. You can find him on Twitter @Mind_OverBatter.
How did the title of this article get past an editor? Yikes
Because it’s a joke?
It’s more a comment on how that reference has been appropriated by a hate group