The Search for the Most Predictable Pitcher
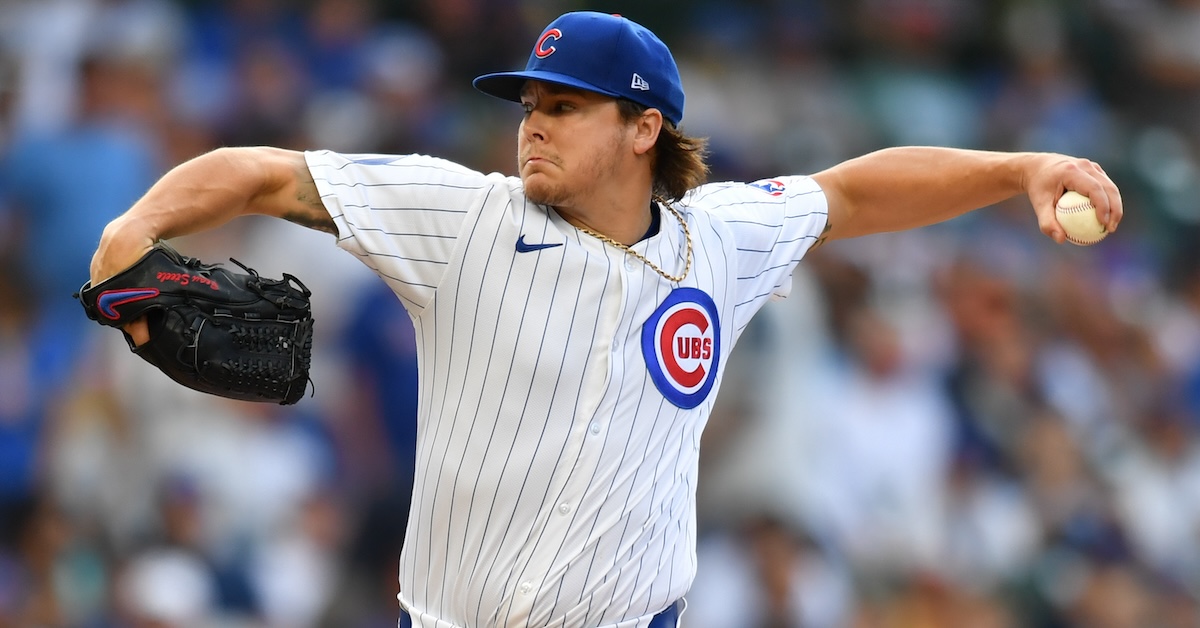
In the pre-PitchCom era, major league teams had more rigorous protocols for protecting their signs than your bank has for securing your account. It wouldn’t surprise me to learn that some teams’ custom PitchCom audio clips are read in a modified pig latin created by a pitching strategy staffer. That the hitter doesn’t know what pitch is coming is considered a huge advantage for the pitcher. And it’s not only pitchers who think so — just ask the 2017 Astros.
Sign-stealing aside, hitters stand in the box pondering which pitch might come hurtling their way mere seconds later. What that pondering looks like depends on the hitter. There’s Nick Castellanos and his “glorified batting practice” approach, in which he looks for the ball and hits it as hard as he can. But there’s also Carlos Correa, who starts his day studying pitcher tendencies in the video room.
For their part, pitchers set the difficulty level on the hitter’s guessing game. That terms like “fastball count” and “pitching backwards” exist tell us that pitchers follow (and, at times, purposefully upend) conventional tactics to sequence their pitches, and believe that certain pitch types are optimal in certain counts. Strategies become standard practices because they’re effective, but an over-reliance on one or two strategies can lead to predictability. Become too predictable and a pitcher effectively sets their opponents’ guessing game on “easy” mode. But does making it easy for the hitter to sit on a certain pitch automatically make the overall task of hitting easier? Does keeping a hitter guessing always ensure effective pitching?
To figure out how predictability factors into a pitcher’s overall strategy, we need to know which pitchers are setting the guessing game on “beginner” and which pitchers set it on “expert.” We can measure the difficulty of anticipating a given pitcher by playing a strictly by-the-numbers guessing game and seeing how it goes. Meaning that for each pitcher, if every hitter stepped up to the plate knowing which pitch the pitcher throws most frequently in all possible counts and guessed accordingly, how often would the hitter be correct? I took the success rates of a hitter using this guessing strategy on each pitcher’s tendencies from this season and mapped those rates to a Predictability Score between 0 and 100, where a score of 100 is the most predictable and a score of 0 is the least predictable. Looking just at pitchers with at least 100 innings pitched so far this season, the top 10 most and least predictable are listed below:
Player | Predictability Score |
---|---|
Justin Steele | 100 |
Kyle Harrison | 89 |
Cristopher Sánchez | 88 |
Kevin Gausman | 86 |
Reynaldo López | 83 |
Hunter Greene | 82 |
Garrett Crochet | 81 |
James Paxton | 81 |
Ryne Nelson | 81 |
MacKenzie Gore | 81 |
Player | Predictability Score |
---|---|
Seth Lugo | 0 |
Michael Lorenzen | 6 |
Miles Mikolas | 6 |
Mitch Keller | 8 |
Nick Martinez | 11 |
Carlos Carrasco | 13 |
Ranger Suárez | 13 |
Kyle Gibson | 14 |
Michael King | 14 |
Aaron Civale | 15 |
The rankings highlight one fairly obvious effect. Pitchers who primarily throw two pitches are far easier to predict than those who throw four or five. Justin Steele throws 60% four-seam fastballs and 30% sliders, with the other 10% split between his sinker, changeup, and curveball. On the other hand, Seth Lugo throws a proverbial kitchen sink composed of 26% curveballs, 25% four-seamers, 19% sinkers, 13% sliders, 6% cutters, and 6% changeups. And yet, their summary stats are eerily similar:
Player | ERA | FIP | K% | BB% | ERA- | FIP- |
---|---|---|---|---|---|---|
Justin Steele | 3.09 | 3.13 | 24.9% | 6.5% | 76 | 78 |
Seth Lugo | 3.19 | 3.44 | 20.8% | 6.1% | 75 | 83 |
Though they employ far different tools and strategies, the outcome is similar. The impressive quality of Steele’s slider, paired with solid fastball command, provides other means for him to keep hitters uncertain even if they correctly guess the pitch type. For Lugo, neither of his main fastball offerings grade out particularly well according to Stuff+, so rather than stick to the conventional wisdom that leads most pitchers to throw fastballs at least 50% of the time, he leans on two above-average breakers amid an array of pitches that he locates well and throws in a variety of counts, effectively setting up a god-mode guessing game for the hitter.
But since declaring two-pitch pitchers easy to predict and five-pitch pitchers more of a mystery isn’t exactly revelatory, let’s go ahead and control for the difficulty of the initial challenge, or how many pitches the hitter is guessing between. Instead of looking just at how often a pitcher throws each pitch in each count, I compared the actual frequency to the expected frequency if the pitcher were equally likely to throw any pitch in any count, i.e. maximum unpredictability. Only pitches with a 10% usage rate or higher were included to limit the scope to offerings a hitter would actually need to keep front of mind while in the box. So even though Lugo throws six pitches, the cutter and the changeup don’t get enough play to make the cut. With that in mind, Lugo is least predictable if there’s a 25% of him throwing any of his four most-used pitches in any count. Taking the absolute difference between his actual count-level usage rates and 25% provides a measure of how far off he is from optimal hitter confusion. Averaging those differences across all counts (weighted by how often he finds himself in each count) and mapping to the same scale used previously gives us a metric for overall comparison across pitchers:
Player | Arsenal Size | Predictability Score |
---|---|---|
Andrew Abbott | 4 | 100 |
Joey Estes | 4 | 100 |
Albert Suárez | 4 | 92 |
Bailey Falter | 4 | 86 |
Kyle Harrison | 3 | 84 |
Yusei Kikuchi | 4 | 83 |
Cristopher Sánchez | 3 | 82 |
Mitchell Parker | 4 | 82 |
Kyle Hendricks | 4 | 75 |
Reynaldo López | 3 | 75 |
Player | Arsenal Size | Predictability Score |
---|---|---|
Patrick Corbin | 3 | 0 |
Luis Severino | 3 | 3 |
Michael Lorenzen | 4 | 3 |
Seth Lugo | 4 | 3 |
Dylan Cease | 2 | 4 |
Miles Mikolas | 4 | 5 |
Hunter Greene | 2 | 5 |
Ben Lively | 3 | 6 |
Garrett Crochet | 2 | 9 |
Mitch Keller | 4 | 9 |
In this framing of the question, Andrew Abbott is the most predictable with his 54% four-seamers, 19% sliders, 16% changeups, and 11% curveballs. Despite his predictability, he rates as above average with an 85 ERA- on the season, though his 116 FIP- casts some doubt on the proceedings and makes an argument for increasing his slider usage, both in the name of keeping hitters guessing and throwing your best pitch more.
Lugo still rates well for his lack of predictability, but perhaps more surprising is two-pitch Dylan Cease slotting in at fifth in the rankings. Cease combines to throw his fastball and slider 90% of the time, but remains tough to predict by splitting that 90% usage almost exactly 50-50. Obviously, the key to Cease’s success with just two pitches is that both pitches grade out extremely well, but throwing them equally often and tunneling them out of a consistent release point amplifies the impact. That said, keeping hitters guessing only goes so far if you have a case of late-career Patrick Corbin on your hands.
Meanwhile, even though five-pitch Zack Wheeler is roughly as predictable as three-pitch Freddy Peralta, the volume of information to process on the way to understanding and acting on those tendencies is greater for Wheeler than Peralta. The size and shape of the game planning process varies based on the size of the arsenal. Since the number of offerings in a pitcher’s inventory sets the initial difficulty level for hitters’ predictions (which they can then toggle up or down based on usage), let’s also look at the raw predictability scores adjusted not for arsenal size, but rather grouped by the number pitches in the utility belt:
Two-Pitch Pitchers | |
---|---|
Player | Predictability Score |
Justin Steele | 100 |
Kevin Gausman | 86 |
Hunter Greene | 82 |
Three-Pitch Pitchers | |
Player | Predictability Score |
Kyle Harrison | 89 |
Cristopher Sánchez | 88 |
Reynaldo López | 83 |
Four-Pitch Pitchers | |
Player | Predictability Score |
Andrew Abbott | 76 |
Joey Estes | 76 |
Albert Suárez | 71 |
Five-Pitch Pitchers | |
Player | Predictability Score |
Logan Gilbert | 39 |
Zack Wheeler | 39 |
Sonny Gray | 38 |
Two-Pitch Pitchers | |
---|---|
Player | Predictability Score |
Dylan Cease | 68 |
Shota Imanaga | 76 |
Ryne Nelson | 81 |
Three-Pitch Pitchers | |
Player | Predictability Score |
Luis Severino | 25 |
Patrick Corbin | 38 |
Kutter Crawford | 38 |
Four-Pitch Pitchers | |
Player | Predictability Score |
Seth Lugo | 0 |
Michael Lorenzen | 6 |
Miles Mikolas | 8 |
Five-Pitch Pitchers | |
Player | Predictability Score |
Nick Martinez | 13 |
Ranger Suárez | 14 |
Dean Kremer | 17 |
Steele and Cease bookend the two-pitch pitchers in terms of prediction success rate, but the gap between Steel and everyone else is in danger of getting sued for trademark infringement by John Fisher. The pitchers in the middle of the two-pitch pitcher leaderboard all situate themselves closer on the spectrum to Cease than Steele. On the one hand, while pitchers with just two primary pitches have made a choice to lean heavily on those offerings and probably wouldn’t do so if they weren’t confident in both of them, mixing both pitches in regularly, at all times, is clearly part of the strategy as well. In fact, many of the two-pitch hurlers are less predictable than their peers with three or even four pitches.
Luis Severino leads the three-pitch group in maximizing guessing game trickery, while Kyle Harrison prefers to rely on stuff over secrecy. The four-pitch crew is led in predictability by Abbott and unpredictability by Lugo. Logan Gilbert is the most predictable amongst the five-pitch crowd, whereas the Nick Martinez code is tougher to crack.
That the least predictable five-pitch pitchers are more predictable than their four-pitch counterparts is interesting, but probably speaks to the luxury inherent in having so many options to deploy. With five pitches, one or two offerings may be reserved for specific situations based on count and hitter handedness. Such a strategy makes a pitcher more predictable, but as long as the pitch remains effective, the trade off is worth it.
Notably absent from every leaderboard so far is an obvious divide in quality when comparing the most predictable to least predictable. Though staying unpredictable is a tool working in the pitcher’s favor, it’s clearly more of a “nice to have” than a “must have.”
We’ve also only measured predictability in the aggregate thus far, giving us a general idea of a pitcher’s strength or weakness in clinging too tightly to a set pattern of behavior. But sometimes even the strongest opponents possess that one hyper-specific weakness, the fatal flaw that when exploited allows a mediocre gamer to triumph over an impossible final boss. Are there specific counts where certain pitchers become so predictable that a hitter could easily exploit their one-note approach?
Since the breadth of pitch selection strategy narrows as the count deepens, it felt logical to start with three-ball and two-strike counts (ignoring full counts because those are a totally separate beast in terms of pitcher approach). In three-ball counts, the pitcher is limited to whatever pitches he feels he can land in the zone, while a pitcher’s advantage in two-strike counts removes the necessity to serve up obvious strikes or anything the hitter might find attractive and instead incentivizes throwing bendy stuff just outside the zone in the hope of getting a swing and miss. Let’s take a look at the leaderboards:
Player | Predictability Score |
---|---|
Andrew Abbott | 92 |
Andrew Heaney | 86 |
Joey Estes | 84 |
Corbin Burnes | 82 |
MacKenzie Gore | 79 |
Player | Predicatability Score |
---|---|
Kyle Gibson | 7 |
Matt Waldron | 13 |
Luis L. Ortiz | 18 |
Carlos Carrasco | 19 |
Ben Lively | 19 |
When pitchers get predictable in three-ball counts, it’s in the really obvious and boring way. They pump fastballs at an obscene rate, and it doesn’t seem to hurt them overall anymore than getting into a three-ball count hurts them in the first place. The aforementioned Abbott — a.k.a., the most predictable pitcher in three-ball counts — throws his four-seamer in 100% of 3-0 counts and 92% of 3-1 counts. Among the top five on the leaderboard, most are already throwing their fastball around 55% of the time. The exception is Corbin Burnes, who takes his typical cutter rate and buys it a gym membership and some protein powder for bulking season, allowing him to grow his cutter usage from 44% overall to 95% in 3-0 counts and 84% in 3-1 counts.
What’s more interesting are the pitchers who manage to be less obvious about their three-ball approach. Like everyone else, Matt Waldron throws more fastballs once he’s got three balls to his name, but that actually serves as a departure from his usual approach, which makes him less predictable. Waldron’s most used pitch is a knuckleball he throws 38% of the time, but in three-ball counts, the knuckleball all but disappears and he splits usage between a four-seamer, sinker, and cutter. His highest usage rate on any pitch is 58% four-seamer in 3-0 counts.
Though Kyle Gibson, the second least predictable pitcher, doesn’t have a knuckleball to ditch, the rest of his strategy for keeping hitters guessing is roughly the same as Waldron’s: throwing multiple fastballs. Of Gibson’s four most used pitches, three are fastballs. So even if he’s worried about landing his sweeper for a strike, he can still make hitters guess between a four-seam, a sinker, and a cutter.
When rating pitchers on predictability with two strikes, there’s a large gap between first place and the rest of the field. Sonny Gray transforms from a pitcher with five pitches that he throws more than 10% of the time and none that he throws more than 25% of the time into a pitcher whose obsession with sweepers rivals the internet’s obsession with Shohei Ohtani’s dog. In 0-2 and 1-2 counts, he throws his sweeper roughly 73% of the time. In 2-2 counts, he chills out with the sweeper a little bit (45% usage) by reintroducing his sinker into the mix (28% usage). It’s hard to argue against his approach since in plate appearances with 0-2, 1-2, or 2-2 counts, hitters are posting wOBAs of .124, .139, and .230, respectively:
Player | Predictability Score |
---|---|
Sonny Gray | 60 |
Joey Estes | 47 |
Jordan Hicks | 47 |
Logan Gilbert | 42 |
Andrew Abbott | 41 |
Player | Predictability Score |
---|---|
Max Fried | 2 |
Miles Mikolas | 3 |
Chris Bassitt | 3 |
Ronel Blanco | 3 |
Seth Lugo | 3 |
On the less predictable side of two-strike counts, Max Fried is the least likely to be overly reliant on the conventional wisdom that calls for pitchers to nibble at the corners with their best breaking pitch. Instead, Fried adheres to another popular baseball cliche and “stays within himself” by keeping his usage roughly the same as his overall numbers. He shaves a few percentage points of usage off his four-seamer and allocates them to his curveball, but otherwise he goes about his business as he would in any other count, using five total pitches at least 10% of the time rather than letting hitters assume they’re getting a curveball or slider out of the zone.
Outside of the counts that impact usage in an intuitive way, one particular pitcher in one particular count stands out as being especially predictable. Joey Estes throws his four-seamer 53% of the time overall, but in 0-1 counts, when he’s already gotten one up on the hitter, he still insists on going to his fastball 66% of the time. It’s his third most frequent fastball count after 3-0 and 3-1. He uses his fastball more frequently in 0-1 counts than he does in 1-0, 2-0, 2-1, or 3-2 counts. I have no guesses for why Estes does this, but I bet opposing hitters see a note about it in their pre-game reports on the nights he’s set to take the mound.
Having now sliced and diced the data a bunch of different ways, we’re at the part of the article where it’s time to sign off with some concluding thoughts. This isn’t the type of article that’s going to end with some neatly wrapped piece of actionable advice to help pitchers hone their craft, and I kind of prefer things that way. Sometimes analytical research is guilty of so thoroughly optimizing strategy that it would be disadvantageous for a team or player to act contrary to the newly discovered, maximally beneficial approach — even if that means a more boring, monochromatic brand of baseball. But sometimes research reveals that more than one strategy can work — that maybe dozens of strategies can work — and that strategies seemingly in opposition with one another can work. What makes a particular strategy optimal depends on the specific team, player, and circumstances in question, and understanding those elements is the key to choosing the best approach.
How cool is it to watch a sport where starting pitchers can be successful throwing two pitches or five pitches? How cool is it to have a sport where both Justin Steele and Dylan Cease can exist and thrive with their disparate usage patterns? How cool is it that some pitchers approach three-ball counts by going all-in on one fastball, while others approach it by going all-in on all the fastballs? How cool is it that baseball has room for Sonny Gray to go ham with sweepers when he gets to two strikes, but also space for Max Fried to roll out his entire arsenal in those same situations? How cool is it to have a quirky little guy hiding out on the Oakland A’s, throwing 66% fastballs in 1-0 counts and hoping no one notices?
If you ask me, it’s all incredibly cool.
Kiri lives in the PNW while contributing part-time to FanGraphs and working full-time as a data scientist. She spent 5 years working as an analyst for multiple MLB organizations. You can find her on Bluesky @kirio.bsky.social.
Good piece. But I was a little surprised that the piece didn’t include batter handedness in addition to the count. Many pitchers reserve one of their pitches for batters of the same handedness, and reserve a different one for batters of opposite handedness. So isn’t every pitcher somewhat more predictable than the article suggests?