A LOBster in Every Pot
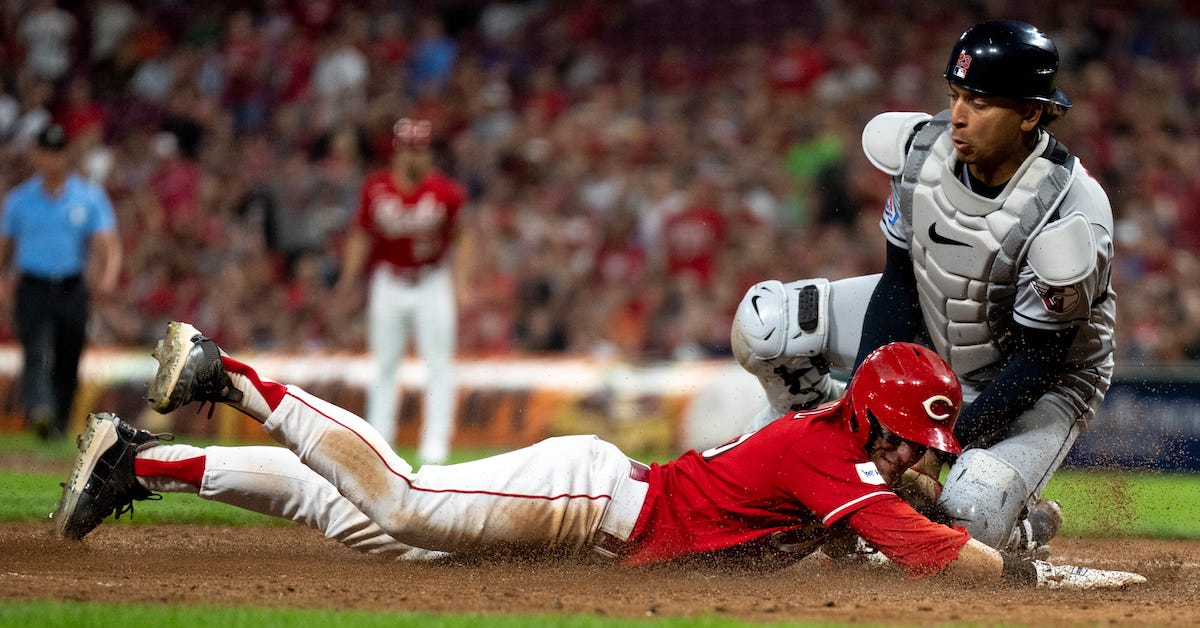
Look, I know that isn’t the line. It’s a chicken in every pot. But I came up with a good lobster pun last week, and I’m writing more about the ins and outs of teams driving home runners from third base, so I decided to go back to the well. You’ll just have to live with it; I’m the one driving the boat here, and as it turns out, it’s a lobster boat.
With the puns are now settled, let’s get down to business. Last week, I chopped up the 2023 season into halves to see how well various statistical indicators correlated with a team’s future ability to cash in their runners. As a recap, strikeout rate had a fairly strong correlation, and not much else did. Quite frankly, though, I wasn’t particularly convinced by that. There just wasn’t enough data. With only 30 observations, it’s too easy for one team to skew things, or at least that’s how it feels in my head.
There’s an easy solution: more data. So I used the same split-half methodology from last week and started chopping past seasons in two. More specifically, I picked the years from 2012-22, excluding the shortened 2020 season. In each case, I followed the same procedure: I split the season in two and noted each team’s offensive statistics in the first half. Then I looked at how efficient each team was at scoring when a runner reached third with less than two outs. I got a much bigger sample this time; 300 observations, which makes it a lot harder for a single outlier to mess things up.
As it turns out, a single outlier might have been messing things up. Over this much larger sample, every major statistical measure of offensive performance did quite “poorly,” at least inasmuch as they predicted very little of a team’s subsequent ability to convert runners on third with less than two outs into runs. In an effort to conserve words and not have to type that long and confusing phrase every time I want to talk about this concept, let’s just call that “conversion rate.”
So yeah… conversion rate is a tricky thing to predict. Here, for your tabular enjoyment, are the correlations between various first-half statistics and second-half conversion rate, as well as r-squared’s in case you don’t feel like doing the math yourself:
Statistic | Correlation | R-Squared |
---|---|---|
BB% | 0.087 | 0.008 |
K% | -0.121 | 0.014 |
ISO | 0.101 | 0.01 |
BABIP | 0.004 | 0 |
AVG | 0.102 | 0.01 |
OBP | 0.136 | 0.018 |
SLG | 0.126 | 0.016 |
wOBA | 0.137 | 0.019 |
wRC+ | 0.124 | 0.015 |
In plain English: blah. None of this is all that interesting. Want to turn your good offensive situations into runs more frequently? Just hit for average… or power… or get on base a lot… or don’t strike out… or just have a generally good offense. The highest correlation between first-half performance and second-half conversion rate is wOBA, which just happens to be the most basic “is your team good at hitting” number available. It does just a hair better than wRC+, which makes sense to me; wRC+ adjusts for park effects, but “did you score a run” and wOBA both don’t. In my future analysis, I decided to throw out BABIP and wRC+; they didn’t seem to be bringing much to the party.
So should you just give up, go the nihilistic route, and say that nothing can help you understand which teams will post the best conversion rates? You could, if you want, in the way that the answer to a ton of baseball questions seems to be “random variance.” But that’s not a particularly satisfying answer, and there are real effects here; better offensive teams really do convert at a higher rate. So I tried a few other methods to try to get at the heart of the problem.
First, I ran two-variable correlations for every combination of two core offensive statistics. I didn’t have a ton of hope that this would give me interesting data, but it’s worth trying something even if you aren’t sure it’ll work just to rule it out. Bad news, though: it didn’t work. I hope you like long, mostly-meaningless tables with far too many data points:
Stat 1 | Stat 2 | Correlation 1 | Correlation 2 | R^2 |
---|---|---|---|---|
BB% | K% | 0.522 | -0.289 | 0.028 |
BB% | ISO | 0.236 | 0.164 | 0.012 |
BB% | AVG | 0.428 | 0.378 | 0.02 |
BB% | OBP | 0.106 | 0.414 | 0.019 |
BB% | SLG | 0.244 | 0.178 | 0.019 |
BB% | wOBA | 0.17 | 0.377 | 0.02 |
K% | ISO | -0.305 | 0.293 | 0.033 |
K% | AVG | -0.184 | 0.161 | 0.016 |
K% | OBP | -0.164 | 0.355 | 0.024 |
K% | SLG | -0.218 | 0.188 | 0.028 |
K% | wOBA | -0.178 | 0.349 | 0.026 |
ISO | AVG | 0.17 | 0.279 | 0.016 |
ISO | OBP | 0.097 | 0.381 | 0.02 |
ISO | SLG | -0.11 | 0.279 | 0.016 |
ISO | wOBA | 0.011 | 0.413 | 0.019 |
AVG | OBP | -0.059 | 0.501 | 0.019 |
AVG | SLG | 0.11 | 0.17 | 0.016 |
AVG | wOBA | -0.048 | 0.459 | 0.019 |
OBP | SLG | 0.317 | 0.089 | 0.02 |
OBP | wOBA | 0.209 | 0.249 | 0.019 |
SLG | wOBA | -0.007 | 0.437 | 0.019 |
These are better, but not by much. And the pairs that explain things the most aren’t the ones I expected. Walk rate and strikeout rate? Strikeout rate and ISO? It makes perfect sense to me that strikeout rate is a key part of the puzzle, but what goes with it doesn’t really track. One small note: because of the way I pulled the data by aggregating results, I couldn’t include fly ball rate, but I’m guessing that ISO is something of a proxy for it, perhaps with other information on hard-hit rate as well.
I didn’t want to stop there, but we’re getting outside of the realm of easily interpretable solutions. A few commenters last week suggested alternatives: dominance analysis, relative weights analysis, principal components analysis, and maybe some others that I missed. I was already familiar with PCA weighting from past experience, but it didn’t really fit with what I was looking for. The other two seemed somewhat promising, but also phenomenally hard to interpret. Then I had an epiphany: If my output is going to be hard to interpret anyway, why not just chuck it into a machine learning algorithm and see what comes out?
Bad news on that front: I chucked it into a variety of machine learning algorithms, and not much came out. Chewing up all that data at once in a multivariate regression did about as well as I did on my own, with a negligible r-squared. Only strikeout rate appeared to be significant, and not very significant at that. I started throwing everything I had at it, including trying to interpret those methods from up above. Relative weights analysis gave ISO, average, and slugging percentage high weights (with slugging having inverse correlation), which sounds like gibberish to me. PCA transformed the data into three uninterpretable principal components, and still only produced an r-squared about as good as using strikeout rate and ISO.
I hate to say it, but I don’t think I can find any clear patterns despite all of this extra data. As an added flourish, the year-to-year correlation between teams’ ability to drive runners home from third with less than two outs is essentially zero, so it’s not like there’s even much evidence that teams have cracked the code. I’m still hopeful that something will shake out, but I have no clue where. Hey, maybe you can help! Here’s a spreadsheet with the data I smashed into various models in the previous section. If you feel like messing around with it, go nuts.
In the end, perhaps the biggest message here is the lack of predictability. You can’t tease out what will happen on the field based on broad aggregates. You can’t forecast what will happen in the biggest moments now based on what happened in the biggest moments in the past. The game today is what matters. No one is doomed to strand those runners, or fated to cash them in. The way you win is by executing each day, not by cashing in on some innate trait that makes you more likely to do the clutch thing. I find that comforting. Even in this age of big data and omnipresent cameras, players doing their thing better is still the most important factor, and who those guys are can and does change from one day to the next.
Ben is a writer at FanGraphs. He can be found on Twitter @_Ben_Clemens.
Super interesting stuff, Ben!