A Simple Method for Evaluating Team Options
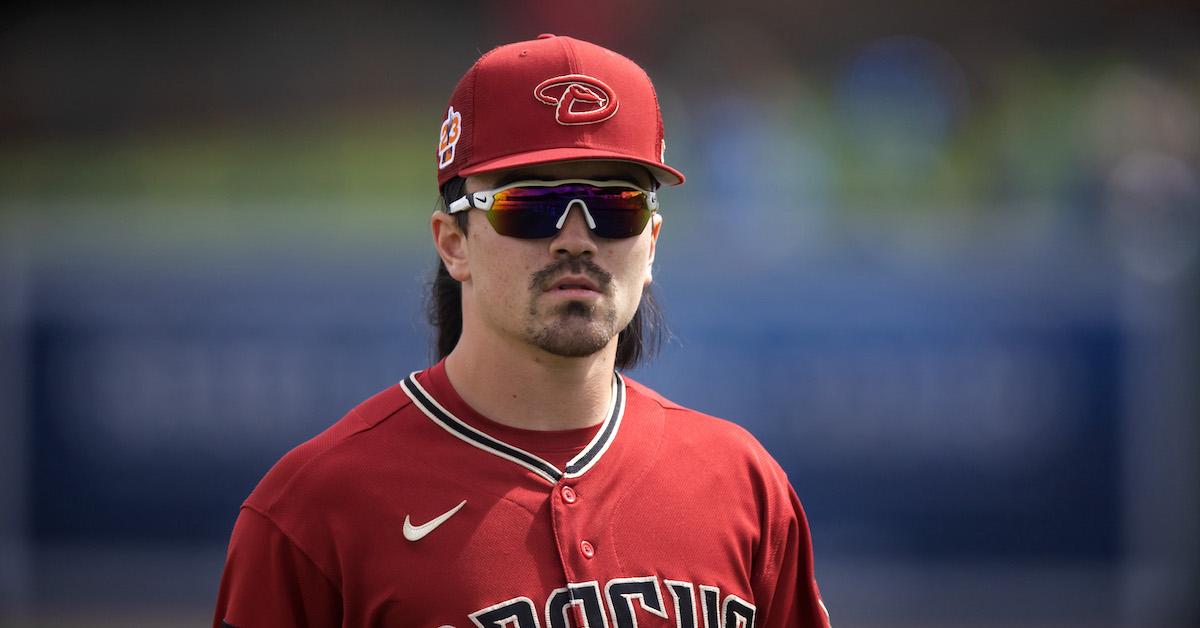
Every time a young star signs a contract extension, we all breathlessly mention the total guarantee. Did you hear Corbin Carroll is getting one hundred and ten million dollars? You could buy a pretty nice house with that, or several nice houses, or live comfortably for the rest of your life and set your kids up to succeed in the bargain. It’s natural to focus on something like that. It is, after all, the main part of the deal.
In almost every one of these extensions, there’s an additional feature: one or more years of team options tacked on to the end of the contract. Our collective analytical view of those tends to be more or less a shrug. “Oh, yeah, and two team options, so that’s nice,” we say, or “well, that makes sense.” I wouldn’t call our evaluations of these options particularly nuanced.
I don’t think that’s going to change on the whole, but the Carroll extension spurred me to at least delve a little deeper into the dollars and cents side of those team options. I’ve already done some work on opt outs from the player perspective, and conveniently enough, I can lift a lot of the mathematical methods from that treatment and use them to evaluate things from the team side.
Today I’m going to model Carroll’s extension, which serves as a template for the general case. The guaranteed part of his deal covers the next eight years, 2023 through 2030. After that, the Diamondbacks can choose to guarantee Carroll a ninth year for $28 million. If they don’t, they owe him a $5 million buyout, and then he’ll become a free agent. That means they need to choose if they want to pay $23 million to keep him in Phoenix for one more year.
Here’s a simplistic decision tree for the Diamondbacks:
2031 Carroll Projected Value | Decision |
---|---|
>$23M | Exercise Option |
<$23M | Decline Option |
Right, obviously, this is easy. When they’re making this decision after the 2030 season, the pluses and minuses will be reasonably straightforward. If Carroll is a star, they’ll gladly keep him. If he has flamed out by then, or is hampered by injuries, or simply never hit the lofty heights this extension assumes he will, they’ll let him walk. Who needs complicated math to figure that one out?
The real question is how likely each of those two choices are now. In 2030, one will be 100% and one will be 0%. But eight years before that point, all we can do is estimate the odds of each happening.
When I’m trying to put probabilities on future outcomes, my favorite tool is a Monte Carlo simulation. That’s a fancy name (they wear tuxedos in Monte Carlo!) for a simple idea. All it means is simulating a random process a ton of times to arrive at your answer.
In this case, the process we’re simulating a ton of times is the passage of time. I’ve abstracted that into some randomly distributed variables. First, we start with a year-zero estimate of Carroll’s talent – I chose 4.5 WAR. From there, I told my computer program to bump his talent randomly every year, using a normal distribution with a standard deviation of 1.4 WAR. This is based on some research I did for that player opt out piece using ZiPS projections, and probably merits a further look at some point.
How much teams are willing to pay for good players in free agency also changes over time, so I added another term: a randomly varying win cost. I had mine start at $8 million per win and increase by $100,000 per year, with random variation around that increase. I added terms for age-related decline (0.4 WAR per year starting at age 30) and also a mean-reverting term that pushes WAR back towards my initial estimate.
All of these terms are up for debate, but the point of this exercise isn’t that I know exactly what all these terms are — it’s that you can define them on your own and then come up with an answer. First, here are the results of trying it my way. The Diamondbacks exercised Carroll’s team option in 69.0% of simulations. He was darn good in those simulations: he projected for an average of 5.5 WAR in 2031 when the team did so.
Throw that projection and my randomly but inexorably increasing estimate of the cost of a win in free agency together, and you get production that would have cost the team $49 million on average in free agency. Since the option always costs them $23 million in salary (the full $28 million minus the $5 million they’ve already committed to paying), that’s a savings of $26 million in cases where the option is exercised.
With these assumptions, the team exercises Carroll’s option 69% of the time, so we can just multiply $26 million by 69% and get that the team projects to come out ahead by around $17.9 million on average in 2031. Sometimes, they come out ahead by zero – they only pay Carroll the buyout they owed him, and he doesn’t play for them. Sometimes, they come out ahead by $26 million (or $30 million, or $18 million — that $26 million is just the average savings versus signing a similar player in free agency). In aggregate, it’s equivalent to $17.9 million of excess value on average.
Just for the sake of completeness, in the 31% of simulations where the team doesn’t pick up Carroll’s option, he’s a lot worse. More specifically, he projects for an average of 0.9 WAR in 2031 in those scenarios. It’s all the bad outcomes, in other words. Conveniently, .69*5.5 plus .31*0.9 works out to 4.1 WAR, which is one year’s aging worse than Carroll’s 4.5 WAR initial projection. It’s a good check that the math worked out. Hey, there are downside scenarios for everyone, particularly nine years out.
That’s a nice cookie for Arizona, and it’s the exact kind of cookie that suits both team and player. Imagine if, instead of asking for a team option, the Diamondbacks just offered Carroll $17.9 million less in guaranteed money. It would save them the same amount of money in expectation, but he’d probably say no. When you’re securing a big bag of money in your first major contract, you want that bag to be big! It’s much easier to cave on things that don’t affect your guarantee, like potential future years after you’ve already made bank.
That’s why team options keep happening: they have meaningful value to teams, and it’s value that players don’t feel bad about surrendering. By adding some conditional sweetener to the deal, teams can come out ahead while still giving players what they care about most: guarantees. It’s a good piece of financial engineering, and it’s no surprise that contracts increasingly use it.
I’ve got good news: if you don’t like my assumptions, you don’t have to stick with them. Just for funsies, I ran a few variations on them to show how sure I am in my estimates. First, I messed around with the mean reversion term, having Carroll regress slightly towards league average instead of more heavily, but towards his own starting projection. I haven’t done enough research to know which of these makes more sense, but my guess is that using initial projection matches reality better, which is why I used it as my base case.
In any case, regressing towards 2 WAR instead of towards 4.5 WAR makes the team less likely to exercise the option (59.9% likely, to be exact). When they do go through with it, though, they get slightly more value, $28.2 million. I think that’s because I relaxed the amount of mean reversion to keep Carroll from just getting dragged down to 2.0 WAR automatically, which doesn’t fit with existing ZiPS projections. In any case, the end result is that the team option projects to be worth $16.9 million to the Diamondbacks, pretty close to what it’s worth in my base case scenario.
What if you think Carroll is worse than I do? I ran it again with a 4.0 WAR initial projection instead of 4.5, this time regressing back to his initial projection rather than league average, per my initial example. This time, the team exercises its option 62.5% of the time, Carroll is a 5.3 WAR player on average in those outcomes, and the aggregate deal is worth $14.9 million in savings to the team averaged across every scenario.
What if you think he’s better? I started him with a 5.0 WAR projection instead, which does basically what you’d expect. It makes Arizona much more likely to exercise its option (74.8% of the time), and makes Carroll better on average when they do (5.8 WAR on average). The net result is that the team option is worth an expected $21.1 million in savings.
Think salaries are never increasing? We can do that too. I removed the cost of a win in free agency variation term entirely, leaving it fixed at $8 million in every simulation. Now the team is slightly less likely to exercise Carroll’s option than in our base case (66.8%), he’s slightly better when they do exercise it (5.6 WAR projection on average), and the option is worth an expected $14.7 million in savings to Arizona.
We could go on moving assumptions this way and that all day, but I think I’ve made my point. An estimate of $15 million to $20 million in expected surplus is roughly right for Carroll’s 2031 team option across a broad array of initial assumptions. If you’re wondering how to think about these tacked-on ends of contract extensions, I think that’s a useful order of magnitude estimate. The teams offering these deals are getting a little bit of value back without lowering the guarantees they offer to players. It’s a good deal across the board.
As always in these types of exercises, if you’re interested in playing with the assumptions yourself, I’ve put the code up for you to use. You can find it here, though I apologize in advance for any extraneous variables in there. I cobbled this together from a few other scripts I already had, and I’d normally spend a little more time making it pretty, but my writing time is occupied with our upcoming positional power rankings. I don’t think it affects the output at all – I ran through the logic plenty of times – but I’m just covering my bases in case you look at the code and find it horrifically ugly. Still, though: free code! And it helps explain something that’s seemingly a part of every contract extension and yet rarely talked about with quantitative rigor. I hope a little sloppiness is okay in that context.
Ben is a writer at FanGraphs. He can be found on Bluesky @benclemens.
Well thought out and VERY well explained method.