Pitcher zStats Entering the Homestretch, Part 1 (Validation)
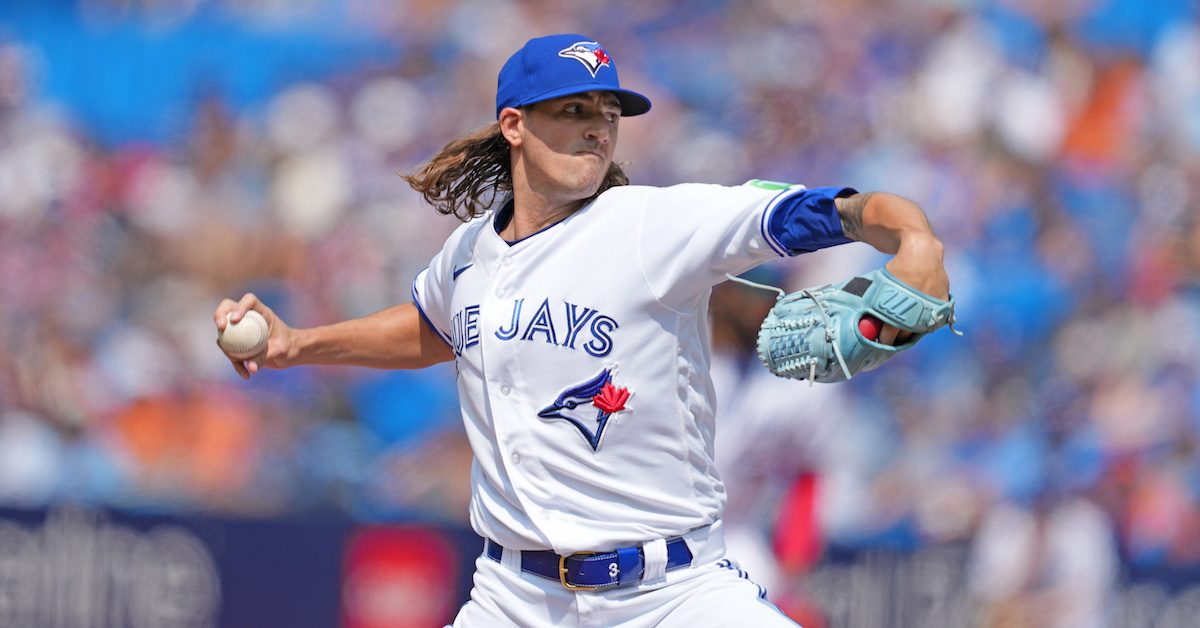
One of the strange things about projecting baseball players is that even results themselves are small samples. Full seasons result in specific numbers that have minimal predictive value, such as BABIP for pitchers. The predictive value isn’t literally zero — individual seasons form much of the basis of projections, whether math-y ones like ZiPS or simply our personal opinions on how good a player is — but we have to develop tools that improve our ability to explain some of these stats. It’s not enough to know that the number of home runs allowed by a pitcher is volatile; we need to know how and why pitchers allow homers beyond a general sense of pitching poorly or being Jordan Lyles.
Data like that which StatCast provides gives us the ability to get at what’s more elemental, such as exit velocities and launch angles and the like — things that are in themselves more predictive than their end products (the number of homers). StatCast has its own implementation of this kind of exercise in its various “x” stats. ZiPS uses slightly different models with a similar purpose, which I’ve dubbed zStats. (I’m going to make you guess what the z stands for!) The differences in the models can be significant. For example, when talking about grounders, balls hit directly toward the second base bag became singles 48.7% of the time from 2012 to ’19, with 51.0% outs and 0.2% doubles. But grounders hit 16 degrees to the “left” of the bag only became hits 10.6% of the time over the same stretch, and toward the second base side, it was 9.8%. ZiPS uses data like sprint speed when calculating hitter BABIP, because how fast a player is has an effect on BABIP and extra-base hits.
ZiPS doesn’t discard actual stats; the models all improve from knowing the actual numbers in addition to the zStats. You can read more on how zStats relate to actual stats here. For those curious about the r-squared values between zStats and real stats for the offensive components, it’s 0.59 for zBABIP, 0.86 for strikeouts, 0.83 for walks, and 0.78 for homers. Those relationships are what make these stats useful for predicting the future. If you can explain 78% of the variance in home run rate between hitters with no information about how many homers they actually hit, you’ve answered a lot of the riddle. All of these numbers correlate better than the actual numbers with future numbers, though a model that uses both zStats and actual ones, as the full model of ZiPS does, is superior to either by themselves.
And why is this important and not just number-spinning? Knowing that changes in walk rates, home run rates, and strikeout rates stabilized far quicker than other stats was an important step forward in player valuation. That’s something that’s useful whether you work for a front office, are a hardcore fan, want to make some fantasy league moves, or even just a regular fan who is rooting for your faves. If we improve our knowledge of the basic molecular structure of a walk or a strikeout, then we can find players who are improving or struggling even more quickly, and provide better answers on why a walk rate or a strikeout rate has changed. This is useful data for me in particular because I obviously do a lot of work with projections, but I’m hoping this type of information is interesting to readers beyond that.
As with any model, the proof of the pudding is in the eating, and there are always some people that question the value of data such as these. So for this run, I’m pitting zStats against the last two months and all new data that obviously could not have been used in the model without a time machine to see how the zStats did compared to reality. I’m not going to do a whole post for this every time, but this is something that, based on the feedback from the last post in June, people really wanted to see the results for.
I addressed hitters earlier this week. Today, we’ll turn our attention to the pitchers. Starting with zBABIP, let’s look at how the numbers have shaken out for the leaders and trailers from back in June. I didn’t include players who faced fewer than 100 batters over the last two months.
Name | HR | zHR | zHR Diff | HR% (6/1) | zHR% (6/1) | HR% Since |
---|---|---|---|---|---|---|
Sonny Gray | 0 | 5.4 | -5.4 | 0.0% | 2.2% | 1.7% |
Zac Gallen | 2 | 7.0 | -5.0 | 0.7% | 2.4% | 4.2% |
Bailey Ober | 3 | 7.0 | -4.0 | 1.9% | 4.5% | 4.3% |
Dane Dunning | 0 | 3.9 | -3.9 | 0.0% | 2.0% | 3.7% |
Justin Steele | 2 | 5.8 | -3.8 | 0.7% | 2.1% | 3.0% |
Michael Wacha | 5 | 8.7 | -3.7 | 2.2% | 3.8% | 2.7% |
Mitch Keller | 7 | 10.6 | -3.6 | 2.3% | 3.5% | 3.9% |
Bryce Elder | 4 | 7.5 | -3.5 | 1.5% | 2.8% | 3.9% |
Nathan Eovaldi | 3 | 6.1 | -3.1 | 1.1% | 2.1% | 2.6% |
Shane McClanahan | 7 | 10.0 | -3.0 | 2.5% | 3.5% | 4.3% |
Adam Wainwright | 3 | 5.9 | -2.9 | 2.4% | 4.7% | 4.6% |
Bryce Miller | 2 | 4.9 | -2.9 | 1.5% | 3.6% | 5.3% |
Logan Allen | 3 | 5.8 | -2.8 | 1.7% | 3.4% | 3.5% |
Alex Lange | 0 | 2.6 | -2.6 | 0.0% | 2.9% | 2.7% |
Patrick Sandoval | 4 | 6.6 | -2.6 | 1.7% | 2.8% | 2.1% |
Merrill Kelly 켈리 | 5 | 7.6 | -2.6 | 2.0% | 3.0% | 3.9% |
Yonny Chirinos | 2 | 4.5 | -2.5 | 1.8% | 4.0% | 5.7% |
Jesús Luzardo | 9 | 11.4 | -2.4 | 3.2% | 4.0% | 2.9% |
Framber Valdez | 6 | 8.3 | -2.3 | 2.1% | 2.9% | 2.4% |
Luis Castillo | 6 | 8.2 | -2.2 | 2.4% | 3.2% | 5.3% |
Jordan Montgomery | 7 | 9.2 | -2.2 | 2.6% | 3.4% | 2.3% |
Name | HR | zHR | zHR Diff | HR% (6/1) | zHR% (6/1) | HR% Since |
---|---|---|---|---|---|---|
Yusei Kikuchi | 15 | 9.7 | 5.3 | 6.2% | 4.0% | 2.6% |
Luis Medina | 10 | 5.3 | 4.7 | 8.3% | 4.4% | 0.9% |
Grayson Rodriguez | 13 | 8.3 | 4.7 | 6.2% | 4.0% | 0.0% |
Ken Waldichuk | 14 | 9.6 | 4.4 | 5.6% | 3.8% | 1.0% |
Julio Urías | 14 | 9.9 | 4.1 | 6.2% | 4.4% | 1.3% |
Ross Stripling | 10 | 6.2 | 3.8 | 6.8% | 4.2% | 3.6% |
Colin Rea | 8 | 4.4 | 3.6 | 4.5% | 2.5% | 4.4% |
Luke Weaver | 11 | 7.5 | 3.5 | 5.9% | 4.0% | 4.5% |
Lance Lynn | 15 | 11.7 | 3.3 | 5.0% | 3.9% | 6.0% |
Tyler Wells | 13 | 9.7 | 3.3 | 5.4% | 4.0% | 5.6% |
Martín Pérez | 10 | 7.0 | 3.0 | 3.7% | 2.6% | 3.8% |
Aaron Nola | 12 | 9.3 | 2.7 | 4.0% | 3.1% | 4.9% |
David Peterson | 8 | 5.4 | 2.6 | 4.4% | 3.0% | 0.9% |
zHR was closer on 16 of 21 overachievers, with a mean absolute error (MAE) of 0.9% vs. 2.1% for the actual home run rate. This is kind of to be expected since zHR is going up against an extremely volatile stat — zHR better have a lower error rate, even with the results themselves being volatile and in a pretty small sample size. We only have 13 of the underachievers returning with enough playing time, thanks to pitchers with high home runs allowed frequently either being injured or quickly losing their jobs. ZiPS was closer on eight of the 13 (MAE 2.0% vs. 2.9%). For all pitchers, not just leaders and trailers, ZiPS had an MAE of 1.4% compared to 2.4% for actual home run rate.
Name | BB | zBB | zBB Diff | BB% (6/1) | zBB% (6/1) | BB% Since |
---|---|---|---|---|---|---|
Framber Valdez | 15 | 24.8 | -9.8 | 5.2% | 8.7% | 7.0% |
Zack Wheeler | 15 | 24.4 | -9.4 | 5.5% | 8.9% | 3.4% |
Tyler Wells | 12 | 20.7 | -8.7 | 5.0% | 8.6% | 9.9% |
Anthony DeSclafani | 9 | 16.9 | -7.9 | 3.3% | 6.2% | 7.5% |
Cristian Javier | 16 | 23.6 | -7.6 | 6.4% | 9.4% | 10.0% |
JP Sears | 11 | 17.0 | -6.0 | 4.5% | 7.0% | 6.4% |
Tanner Bibee | 10 | 15.8 | -5.8 | 6.3% | 10.0% | 9.0% |
Dean Kremer | 17 | 22.6 | -5.6 | 6.7% | 8.9% | 7.9% |
Zac Gallen | 16 | 21.4 | -5.4 | 5.5% | 7.4% | 4.9% |
Nathan Eovaldi | 14 | 19.4 | -5.4 | 4.9% | 6.8% | 10.3% |
Matt Brash | 9 | 14.3 | -5.3 | 8.7% | 13.8% | 10.7% |
Jesús Luzardo | 20 | 25.2 | -5.2 | 7.1% | 8.9% | 6.5% |
J.P. France | 8 | 12.9 | -4.9 | 6.8% | 11.0% | 7.6% |
Wade Miley | 9 | 13.9 | -4.9 | 5.3% | 8.1% | 10.6% |
Jordan Montgomery | 17 | 21.6 | -4.6 | 6.3% | 8.0% | 7.2% |
Taj Bradley | 5 | 9.6 | -4.6 | 4.1% | 7.9% | 10.1% |
Zach Eflin | 8 | 12.5 | -4.5 | 3.4% | 5.4% | 3.3% |
Josh Winckowski | 7 | 11.5 | -4.5 | 5.1% | 8.4% | 8.7% |
Yennier Cano | 1 | 5.5 | -4.5 | 1.0% | 5.5% | 7.3% |
Bryce Miller | 3 | 7.3 | -4.3 | 2.2% | 5.4% | 6.7% |
Name | BB | zBB | zBB Diff | BB% (6/1) | zBB% (6/1) | BB% Since |
---|---|---|---|---|---|---|
Alek Manoah | 41 | 27.8 | 13.2 | 15.0% | 10.2% | 12.2% |
Tyler Anderson | 25 | 16.5 | 8.5 | 10.5% | 6.9% | 8.4% |
Shane McClanahan | 27 | 18.8 | 8.2 | 9.6% | 6.7% | 7.5% |
Spencer Strider | 22 | 14.0 | 8.0 | 8.6% | 5.5% | 6.6% |
MacKenzie Gore | 28 | 20.3 | 7.7 | 11.3% | 8.2% | 9.1% |
Tanner Scott | 14 | 8.0 | 6.0 | 13.3% | 7.6% | 7.2% |
Chris Bassitt | 26 | 20.1 | 5.9 | 9.4% | 7.3% | 6.3% |
Sandy Alcantara | 25 | 19.4 | 5.6 | 8.5% | 6.6% | 4.0% |
Edward Cabrera | 35 | 29.4 | 5.6 | 15.1% | 12.7% | 16.2% |
Johan Oviedo | 30 | 24.5 | 5.5 | 11.2% | 9.1% | 8.0% |
Ken Waldichuk | 32 | 26.5 | 5.5 | 12.9% | 10.7% | 12.0% |
Sean Manaea | 19 | 13.7 | 5.3 | 11.4% | 8.2% | 5.9% |
Taijuan Walker | 26 | 20.8 | 5.2 | 10.6% | 8.4% | 8.8% |
Gerrit Cole | 26 | 20.9 | 5.1 | 8.6% | 7.0% | 4.7% |
Daniel Bard | 11 | 5.9 | 5.1 | 17.5% | 9.3% | 22.0% |
Sam Moll | 13 | 7.9 | 5.1 | 15.9% | 9.7% | 5.7% |
Martín Pérez | 20 | 15.0 | 5.0 | 7.4% | 5.6% | 9.9% |
Merrill Kelly | 25 | 20.1 | 4.9 | 9.9% | 8.0% | 8.3% |
zBB beat actual walk rate on 14 of the 20 returning overachievers and 14 of the 18 underachievers. The MAEs for the zStats were both lower as well (1.9% vs. 2.9% and 2.4% vs. 3.3%). The same pattern held true for all pitchers, at 2.1% vs. 3.1%.
Name | SO | zSO | zSO Diff | SO% (6/1) | zSO% (6/1) | SO% Since |
---|---|---|---|---|---|---|
Kevin Gausman | 100 | 79.9 | 20.1 | 32.9% | 26.3% | 33.7% |
Mitch Keller | 93 | 78.6 | 14.4 | 30.4% | 25.7% | 19.7% |
Merrill Kelly | 69 | 55.6 | 13.4 | 27.4% | 22.1% | 24.0% |
Logan Gilbert | 73 | 59.9 | 13.1 | 28.7% | 23.6% | 22.0% |
Zac Gallen | 82 | 69.5 | 12.5 | 28.4% | 24.1% | 24.4% |
Framber Valdez | 77 | 64.6 | 12.4 | 26.9% | 22.6% | 23.4% |
Andrew Heaney | 55 | 42.7 | 12.3 | 24.3% | 18.9% | 24.9% |
Taj Bradley | 42 | 31.0 | 11.0 | 34.4% | 25.4% | 27.4% |
Alexis Díaz | 41 | 30.7 | 10.3 | 48.8% | 36.6% | 25.8% |
Joe Ryan | 76 | 66.0 | 10.0 | 29.1% | 25.3% | 29.0% |
Clarke Schmidt | 65 | 55.0 | 10.0 | 26.0% | 22.0% | 18.4% |
Edward Cabrera | 66 | 56.1 | 9.9 | 28.4% | 24.2% | 25.7% |
Seth Lugo | 38 | 29.1 | 8.9 | 21.3% | 16.3% | 25.1% |
Yennier Cano | 30 | 21.2 | 8.8 | 30.0% | 21.2% | 18.3% |
Lance Lynn | 76 | 67.3 | 8.7 | 25.1% | 22.2% | 28.7% |
Kyle Freeland | 43 | 34.3 | 8.7 | 16.1% | 12.8% | 13.1% |
Grayson Rodriguez | 56 | 47.4 | 8.6 | 26.5% | 22.5% | 21.2% |
Hunter Brown | 74 | 65.6 | 8.4 | 28.8% | 25.5% | 24.0% |
Gerrit Cole | 79 | 70.9 | 8.1 | 26.2% | 23.6% | 27.3% |
Name | SO | zSO | zSO Diff | SO% (6/1) | zSO% (6/1) | SO% Since |
---|---|---|---|---|---|---|
Shane Bieber | 53 | 70.4 | -17.4 | 16.9% | 22.4% | 24.0% |
Tyler Anderson | 33 | 48.8 | -15.8 | 13.8% | 20.4% | 23.3% |
Patrick Corbin | 42 | 56.8 | -14.8 | 14.0% | 18.9% | 16.5% |
Patrick Sandoval | 36 | 49.2 | -13.2 | 15.1% | 20.6% | 23.4% |
Johan Oviedo | 53 | 64.5 | -11.5 | 19.8% | 24.1% | 21.4% |
Shane McClanahan | 82 | 92.9 | -10.9 | 29.1% | 32.9% | 20.9% |
Nick Martinez | 37 | 47.8 | -10.8 | 19.4% | 25.0% | 24.2% |
Ian Gibaut | 22 | 31.3 | -9.3 | 20.6% | 29.3% | 20.3% |
Jon Gray | 46 | 54.6 | -8.6 | 19.9% | 23.6% | 20.7% |
Gregory Santos | 26 | 34.1 | -8.1 | 21.3% | 27.9% | 25.2% |
Sandy Alcantara | 60 | 68.0 | -8.0 | 20.5% | 23.2% | 20.1% |
Emmanuel Clase | 20 | 28.0 | -8.0 | 17.7% | 24.8% | 27.2% |
Corbin Burnes | 59 | 66.8 | -7.8 | 22.3% | 25.2% | 27.4% |
J.P. France | 26 | 33.4 | -7.4 | 22.2% | 28.6% | 15.2% |
For the overachievers, zSO was closer to results than the actual strikeouts were for 13 of the 19 players (3.7% vs. 5.4%). I was concerned about underachievers, given that a lot of the pitchers involved had injury questions, but ZiPS still eked out wins here both in roughly being closer (eight of 14) and MAE (4.4% vs. 4.9%). For all pitchers, the MAE was 3.9% vs. 5.1%. Since I’ve been a long time Kevin Gausman obsessee, I’m happy to have zSO miss on that one.
Name | FIP ER | zFIP ER | zFIP ER Diff | FIP (6/1) | zFIP (6/1) | FIP Since |
---|---|---|---|---|---|---|
Zac Gallen | 16.9 | 27.1 | -10.3 | 2.09 | 3.36 | 4.18 |
Sonny Gray | 14.7 | 23.4 | -8.6 | 2.20 | 3.49 | 3.31 |
Framber Valdez | 23.5 | 31.0 | -7.5 | 2.94 | 3.88 | 3.68 |
Kevin Gausman | 19.8 | 26.3 | -6.4 | 2.38 | 3.15 | 3.08 |
Bailey Ober | 14.5 | 20.4 | -5.8 | 3.24 | 4.54 | 4.22 |
Brad Hand | 4.5 | 10.0 | -5.4 | 2.01 | 4.42 | 5.95 |
Nathan Eovaldi | 21.0 | 26.4 | -5.4 | 2.54 | 3.19 | 4.32 |
Bryce Miller | 10.8 | 16.2 | -5.3 | 2.71 | 4.04 | 4.88 |
Yennier Cano | 4.6 | 9.9 | -5.3 | 1.43 | 3.06 | 4.30 |
Mitch Keller | 23.7 | 28.8 | -5.1 | 2.86 | 3.48 | 5.11 |
Chris Stratton | 8.9 | 13.6 | -4.7 | 2.58 | 3.94 | 3.34 |
Michael Wacha | 22.6 | 27.2 | -4.6 | 3.55 | 4.27 | 4.12 |
Bryce Elder | 25.0 | 29.0 | -3.9 | 3.43 | 3.97 | 5.38 |
Adam Wainwright | 13.1 | 16.9 | -3.8 | 4.47 | 5.77 | 6.52 |
Dane Dunning | 15.1 | 18.8 | -3.7 | 2.83 | 3.53 | 4.89 |
Fernando Cruz | 4.9 | 8.5 | -3.6 | 2.84 | 4.89 | 3.17 |
Cristian Javier | 27.0 | 30.6 | -3.5 | 3.82 | 4.32 | 5.41 |
Logan Allen | 13.0 | 16.3 | -3.4 | 2.94 | 3.71 | 4.83 |
Merrill Kelly | 24.2 | 27.6 | -3.4 | 3.42 | 3.90 | 4.57 |
Andrew Wantz | 7.9 | 11.1 | -3.2 | 3.29 | 4.62 | 9.27 |
Dauri Moreta | 8.4 | 11.6 | -3.2 | 3.01 | 4.16 | 3.61 |
Josh Hader | 5.8 | 9.0 | -3.2 | 2.35 | 3.63 | 2.24 |
Mark Leiter Jr. | 8.6 | 11.8 | -3.1 | 3.42 | 4.67 | 3.38 |
Emilio Pagan | 8.1 | 11.2 | -3.1 | 3.04 | 4.20 | 3.97 |
Name | FIP ER | zFIP ER | zFIP ER Diff | FIP (6/1) | zFIP (6/1) | FIP Since |
---|---|---|---|---|---|---|
Chris Bassitt | 38.5 | 32.1 | 6.4 | 5.22 | 4.35 | 4.01 |
Corbin Burnes | 32.2 | 25.1 | 7.1 | 4.55 | 3.54 | 3.32 |
Taijuan Walker | 34.4 | 28.4 | 6.0 | 5.40 | 4.46 | 3.86 |
Patrick Corbin | 37.3 | 30.8 | 6.5 | 4.96 | 4.09 | 5.45 |
Aaron Nola | 36.5 | 29.8 | 6.7 | 4.40 | 3.59 | 4.23 |
Lance Lynn | 39.4 | 33.7 | 5.8 | 5.27 | 4.50 | 5.32 |
Yusei Kikuchi | 37.4 | 28.6 | 8.8 | 5.97 | 4.57 | 3.30 |
Colin Rea | 25.3 | 19.4 | 5.9 | 5.37 | 4.12 | 4.89 |
Luke Weaver | 26.2 | 20.0 | 6.2 | 5.40 | 4.12 | 6.21 |
Jon Gray | 29.7 | 23.4 | 6.3 | 4.64 | 3.65 | 3.91 |
Tyler Anderson | 31.4 | 24.1 | 7.3 | 5.36 | 4.12 | 3.39 |
Martín Pérez | 34.0 | 25.4 | 8.6 | 5.01 | 3.74 | 5.65 |
Ken Waldichuk | 39.5 | 31.0 | 8.5 | 7.02 | 5.51 | 3.50 |
Shane Bieber | 34.5 | 28.3 | 6.2 | 4.14 | 3.40 | 4.13 |
Julio Urías | 32.6 | 25.7 | 7.0 | 5.31 | 4.17 | 3.19 |
Ross Stripling | 24.1 | 17.9 | 6.3 | 6.72 | 4.98 | 3.64 |
Alek Manoah | 40.2 | 31.5 | 8.6 | 6.27 | 4.92 | 6.08 |
This is where we tie everything together, and it’s not an easy matchup considering one of the benefits of FIP is supposed to be its lack of volatility. zFIP was closer on 21 of 24 overachievers (MAE of 1.04 vs. 1.61) and on nine of 17 underachievers (MAE of 1.01 vs. 1.23). For all pitchers, the MAE was 1.03 for zFIP, 1.52 for FIP).
On a year-to-year basis, looking at players with 200 TBF in consecutive seasons (about 1500 players), ZiPS edges out the real stats in all four categories. The MAE edges are 0.9% vs. 1.1% in homer rate, 1.6% vs. 1.8% in strikeout rate, 3.3% vs. 3.5% in walk rate, and 0.72 against 0.82 in FIP. Naturally, zStats combined with the actual ones does even better than either category individually. Based on history, I modeled the ideal linear mix of zStats and actual numbers in predicting next year’s stats. For homers, it’s 92% zStats vs. 8% actual. While that might seem shocking, it’s simply due to the fact that home runs for pitchers are incredibly volatile, which is why xFIP improves on FIP just by assuming that homers are given up at a league-average rate by all pitchers, an intentionally preposterous scenario. For walks, it’s 72% zBB vs. actual. For strikeouts, the tally comes in at 57% zSO, 43% actual. As one final reminder, zStats contain no element of regression toward the mean; it only evaluates the Statcast/plate discipline data for the time periods in question.
Tomorrow, I’ll have the up-to-date zStat leaderboards for the pitchers.
Dan Szymborski is a senior writer for FanGraphs and the developer of the ZiPS projection system. He was a writer for ESPN.com from 2010-2018, a regular guest on a number of radio shows and podcasts, and a voting BBWAA member. He also maintains a terrible Twitter account at @DSzymborski.
One takeaway I had is that overperformers are more likely to regress down than underachievers are likely to regress up. Do other folks have this sense? My hypothesis is underachievers may have latent issues (injuries, mechanics, etc.) that cause the underperformance; for overperformers, there is no corresponding spinach-in-a-can to make them do better.