Checking Out 2022 zStats for Hitters After Two Months of Play
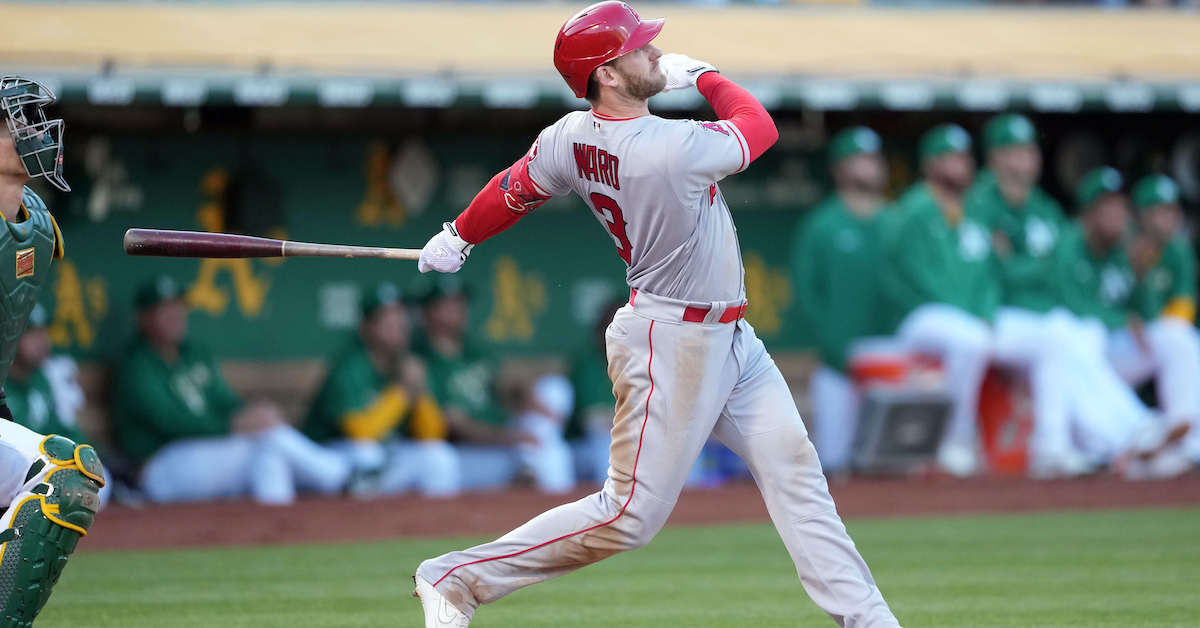
As anyone who does a lot of work with projections could likely tell you, one of the most annoying things about modeling future performance is that results themselves are a small sample size. Individual seasons, even full ones over 162 games, still feature results that are not very predictive, such as a hitter or a pitcher with a BABIP low or high enough to be practically unsustainable. For example, if Luis Arraez finishes the season hitting .350, we don’t actually know that a median projection of .350 was, in fact, the correct projection going into the season. There’s no divine baseball exchecquer to swoop in and let you know if he was “actually” a .350 hitter who did what he was supposed to, a .320 hitter who got lucky, or even a .380 hitter who suffered misfortune. If you flip heads on a coin eight times out of ten and have no reason to believe you have a special coin-flipping ability, you’ll eventually see the split approach 50/50 given a sufficiently large number of coin flips. Convergence in probability is a fairly large academic area that we thankfully do not need to go into here. But for most things in baseball, you never actually get enough coin flips to see this happen. The boundaries of a season are quite strict.
What does this have to do with projections? This volatile data becomes the source of future predictions, and one of the things done in projections is to find things that are not only as predictive as the ordinary stats, but also more predictive based on fewer plate appearances or batters faced. Imagine, for example, if body mass index was a wonderful predictor of isolated power. It would be a highly useful one, as changes to that over the course of a season are bound to be rather small. Underlying reasons for performance tend to be more stable than the results, which is why ERA is more volatile than strikeout rate and why strikeout rate is more volatile than plate discipline stats that result in strikeout rate.
MLB’s own method comes with an x before the stat, whereas what ZiPS uses internally has a z. I’ll let you guess what it stands for! I’ve written more about this stuff in various places such as here and here, so let’s get right to the data for the first two months of the MLB season.
Name | BABIP | zBABIP | zBABIP Diff |
---|---|---|---|
Gilberto Celestino | .439 | .313 | .126 |
J.D. Martinez | .458 | .355 | .103 |
Mike Brosseau | .341 | .239 | .102 |
Taylor Ward | .391 | .290 | .101 |
William Contreras | .348 | .254 | .094 |
Tucker Barnhart | .354 | .261 | .093 |
Manny Machado | .366 | .277 | .089 |
Tyler Stephenson | .396 | .310 | .086 |
Miguel Cabrera | .397 | .317 | .080 |
Luis González | .379 | .301 | .078 |
Ronald Acuña Jr. | .426 | .348 | .078 |
Seiya Suzuki | .341 | .263 | .078 |
Max Stassi | .324 | .247 | .077 |
Mike Moustakas | .242 | .166 | .076 |
Paul Goldschmidt | .395 | .323 | .072 |
ZiPS actually thinks that Taylor Ward will keep quite a lot of the power and plate discipline, but it’s much less confident about his .391 BABIP, which is fueling his current .333 batting average. So there are valid reasons to at least expect some drift downward, even though ZiPS is overall projecting him, when healthy, to stay a big plus contributor (a 143 wRC+ the rest of the season).
One thing to remember is that zBABIP is not as strong an indicator as some of the other z numbers (like zSO for pitchers), and there’s quite a lot of actual BABIP that goes into any prediction. Paul Goldschmidt is a great example of this. He has a historical tendency to exceed his zBABIP, something ZiPS knows about, so full-fat ZiPS projects him at a .340 BABIP over the rest of the season, not .323 (the simpler in-season model is .332). One fun quirk of a player not on this list is J.D Davis; at a .342 BABIP, ZiPS thinks he’s actually underperforming, and his .371 zBABIP is the highest in baseball. He’s been crushing the ball!
Name | BABIP | zBABIP | zBABIP Diff |
---|---|---|---|
Cal Raleigh | .160 | .294 | -.134 |
Andrew Velazquez | .238 | .337 | -.099 |
Taylor Walls | .198 | .290 | -.092 |
Martín Maldonado | .160 | .252 | -.092 |
Christian Walker | .184 | .275 | -.091 |
Leury García | .219 | .309 | -.090 |
Robinson Canó | .192 | .278 | -.086 |
Christian Arroyo | .197 | .282 | -.085 |
Cristian Pache | .207 | .291 | -.084 |
Kyle Higashioka | .203 | .286 | -.083 |
Corey Seager | .227 | .307 | -.080 |
Joc Pederson | .247 | .326 | -.079 |
Kevin Kiermaier | .257 | .332 | -.075 |
Vidal Bruján | .169 | .242 | -.073 |
Mike Zunino | .190 | .263 | -.073 |
Generally speaking, BABIPs below the .220 range or so tend to be very hard to sustain over long periods. You can see why when you look at how pitchers hit. Almost never selected for their offensive abilities — especially now given that pitchers no longer hit — they historically have had a BABIP in the .210–.240 range, depending on the season. Now, a lot of these zBABIPs still aren’t all that exciting, such as Martín Maldonado’s .252 or Vidal Bruján’s .242, but they’re much better than their performances so far. Hopefully you weren’t foolish enough to get off the Corey Seager train! But for those Joc Pederson enthusiasts, he’s actually underperforming in at least one aspect, something that’s hard to usually find on guy with a .937 OPS.
Name | SLG | zSLG | zSLG Diff |
---|---|---|---|
William Contreras | .684 | .526 | .158 |
Paul Goldschmidt | .602 | .475 | .127 |
Gilberto Celestino | .384 | .264 | .120 |
Jose Miranda | .426 | .323 | .103 |
Odubel Herrera | .495 | .397 | .098 |
Manny Machado | .543 | .445 | .098 |
Edwin Rios | .500 | .404 | .096 |
Randal Grichuk | .391 | .299 | .092 |
Luis González | .441 | .351 | .090 |
Tyler Stephenson | .475 | .386 | .089 |
Aaron Judge | .675 | .594 | .081 |
Taylor Ward | .644 | .565 | .079 |
Mike Brosseau | .412 | .333 | .079 |
Chad Pinder | .399 | .326 | .073 |
Matt Reynolds | .402 | .329 | .073 |
Just to start, zSLG’s historical r^2 for seasons of at least 300 PA is 0.71. Don’t be disappointed about seeing Ward here again; as I said at the top, he still has quite a robust power number here. If I told you before the season, using a time machine in a very odd way, that Taylor Ward would hit under .300 but slug .565, would you actually believe me? (I am aware that convincing you that I’m a time traveler would probably be even more difficult.) Similarly, Aaron Judge’s numbers still look elite, just not quite to the extent of his destruction of the league. On the other hand, ZiPS sees all other current .600 sluggers with less than a 30-point drop to zSLG (Yordan Alvarez, Bryce Harper, José Ramírez, Rafael Devers, Mike Trout). You may notice that Joc Pederson is not on this list; ZiPS thinks he should be slugging .629!
Name | SLG | zSLG | zSLG Diff |
---|---|---|---|
Kyle Higashioka | .207 | .483 | -.276 |
Ernie Clement | .260 | .443 | -.183 |
Christian Arroyo | .329 | .506 | -.177 |
Max Muncy | .263 | .437 | -.174 |
Leury García | .257 | .423 | -.166 |
Robinson Canó | .189 | .354 | -.165 |
Sam Hilliard | .297 | .460 | -.163 |
Yasmani Grandal | .213 | .365 | -.152 |
TJ Friedl | .239 | .390 | -.151 |
Christian Bethancourt 베탄코트 | .324 | .469 | -.145 |
Jeimer Candelario | .319 | .463 | -.144 |
Jason Heyward | .272 | .409 | -.137 |
J.D. Davis | .349 | .481 | -.132 |
Ryan Jeffers | .310 | .441 | -.131 |
Franmil Reyes | .278 | .406 | -.128 |
ZiPS still loves you, Kyle Higashioka!
Seeing Leury Garcia on both underachiever lists makes me wonder if I’ve been too harsh in mocking the White Sox for their aggressive use of him. Robinson Canó’s double appearance is enough to make me think that a lousy team should be looking at him, so long as if said team doesn’t do a Carlos Santana/Royals thing and use him to block someone far more interesting. Just missing the list was Joey Gallo, though I’m not sure that even at a .451 zSLG, he’s all that exciting, which is a complete sea change from a year ago.
zBB and zSO for hitters is highly predictive, with r^2 numbers of 0.814 and 0.858, respectively, which isn’t that surprising since walks and strikeouts are quite predictive by themselves, at least compared to other hitters’ numbers. Rather than focus on the overachievers and underachievers, I’ll just post the leaders and trailers here. With the normal numbers being so useful, ZiPS mostly uses these to shade the numbers toward the (hopefully) right direction.
Name | BB% | zBB | Diff |
---|---|---|---|
Juan Soto | 18.0% | 18.1% | -0.1% |
Max Muncy | 20.2% | 17.5% | 2.8% |
Taylor Ward | 16.5% | 16.0% | 0.4% |
Yandy Díaz | 18.3% | 15.4% | 2.9% |
Ji-Man Choi | 13.2% | 15.3% | -2.1% |
Alex Bregman | 14.3% | 15.0% | -0.7% |
Mike Yastrzemski | 14.1% | 14.5% | -0.5% |
Will Smith | 13.7% | 14.5% | -0.7% |
Kyle Schwarber | 15.0% | 14.4% | 0.6% |
Victor Caratini | 11.2% | 14.4% | -3.1% |
Trent Grisham | 11.8% | 14.3% | -2.5% |
Mike Trout | 12.6% | 14.2% | -1.6% |
Anthony Rendon | 12.1% | 13.7% | -1.6% |
Brandon Belt | 13.3% | 13.6% | -0.2% |
Yordan Alvarez | 13.0% | 13.4% | -0.3% |
Name | BB% | zBB | Diff |
---|---|---|---|
Edmundo Sosa | 2.4% | 0.1% | 2.3% |
Francisco Mejía | 1.1% | 0.4% | 0.7% |
Jorge Alfaro | 5.0% | 1.8% | 3.1% |
Jose Trevino | 7.3% | 2.6% | 4.7% |
José Iglesias | 3.9% | 2.7% | 1.2% |
Albert Almora Jr. | 2.4% | 2.9% | -0.5% |
Reese McGuire | 3.8% | 3.0% | 0.8% |
Maikel Franco | 3.5% | 3.2% | 0.3% |
Amed Rosario | 5.0% | 3.3% | 1.6% |
Harold Ramirez | 4.8% | 3.4% | 1.5% |
Yan Gomes | 2.7% | 3.4% | -0.7% |
Willi Castro | 3.8% | 3.4% | 0.5% |
Jorge Mateo | 3.2% | 3.5% | -0.2% |
Tim Anderson | 3.5% | 3.5% | -0.1% |
Nico Hoerner | 2.7% | 3.6% | -0.9% |
I’m shocked — shocked — to see Juan Soto on top of a plate discipline list. Jose Trevino is the third-biggest overachiever in walk rate, so don’t get too attached to that on-base percentage (he’s also a BABIP overachiever of 22 points). ZiPS is completely befuddled how Edmundo Sosa has even drawn two walks given his abysmal contact and swing numbers.
Name | SO% | zSO | Diff |
---|---|---|---|
Steven Kwan | 7.2% | 8.4% | -1.2% |
Joey Wendle | 11.3% | 8.6% | 2.8% |
Wander Franco | 10.5% | 8.8% | 1.7% |
Luis Arraez | 8.3% | 8.8% | -0.5% |
Miguel Rojas | 10.0% | 10.0% | 0.0% |
José Ramírez | 7.0% | 10.2% | -3.2% |
Mauricio Dubón | 5.2% | 10.5% | -5.3% |
Alex Verdugo | 10.4% | 10.8% | -0.4% |
Keibert Ruiz | 9.4% | 11.0% | -1.5% |
Adam Frazier | 12.2% | 11.0% | 1.2% |
Alejandro Kirk | 9.3% | 11.0% | -1.7% |
Michael Brantley | 10.4% | 11.2% | -0.8% |
Jeff McNeil | 11.8% | 11.4% | 0.4% |
Yandy Díaz | 8.9% | 12.0% | -3.1% |
Kyle Isbel | 23.4% | 12.1% | 11.3% |
Name | SO% | zSO | Diff |
---|---|---|---|
Eli White | 36.4% | 43.8% | -7.4% |
Brett Phillips | 42.1% | 41.9% | 0.2% |
Keston Hiura | 45.7% | 41.0% | 4.8% |
Joey Bart | 45.4% | 39.6% | 5.8% |
Chris Taylor | 34.3% | 37.7% | -3.4% |
Trevor Larnach | 32.1% | 37.1% | -5.0% |
Edwin Ríos | 39.1% | 37.0% | 2.1% |
Mike Zunino | 37.5% | 36.0% | 1.5% |
Travis Demeritte | 33.3% | 35.5% | -2.1% |
Javier Báez | 26.8% | 35.3% | -8.5% |
Luke Voit | 33.3% | 34.8% | -1.5% |
Franmil Reyes | 39.3% | 34.8% | 4.5% |
Austin Slater | 27.9% | 34.6% | -6.7% |
Jarred Kelenic | 37.5% | 34.5% | 3.0% |
Joey Gallo | 38.1% | 34.3% | 3.8% |
Not a whole lot of major surprises here. Steven Kwan may be slumping, but his ability to avoid strikeouts is real and should keep his batting average from bottoming out, which ought to be enough to stick in Cleveland given the general depth in the outfield there. Seeing Kyle Isbel on this list makes me wonder if it’s an approach/coaching problem with him. It’s unusual to see high contact rate guys — and with an 87.8% contact rate, that’s what he’s been this year — with so many actual strikeouts. That might be something worth digging into further.
Coming on Friday: I turn my attention to pitchers.
Dan Szymborski is a senior writer for FanGraphs and the developer of the ZiPS projection system. He was a writer for ESPN.com from 2010-2018, a regular guest on a number of radio shows and podcasts, and a voting BBWAA member. He also maintains a terrible Twitter account at @DSzymborski.
A player on the Royals getting coached improperly? I’m shocked, I tell you, shocked.