Prospect Week Primer
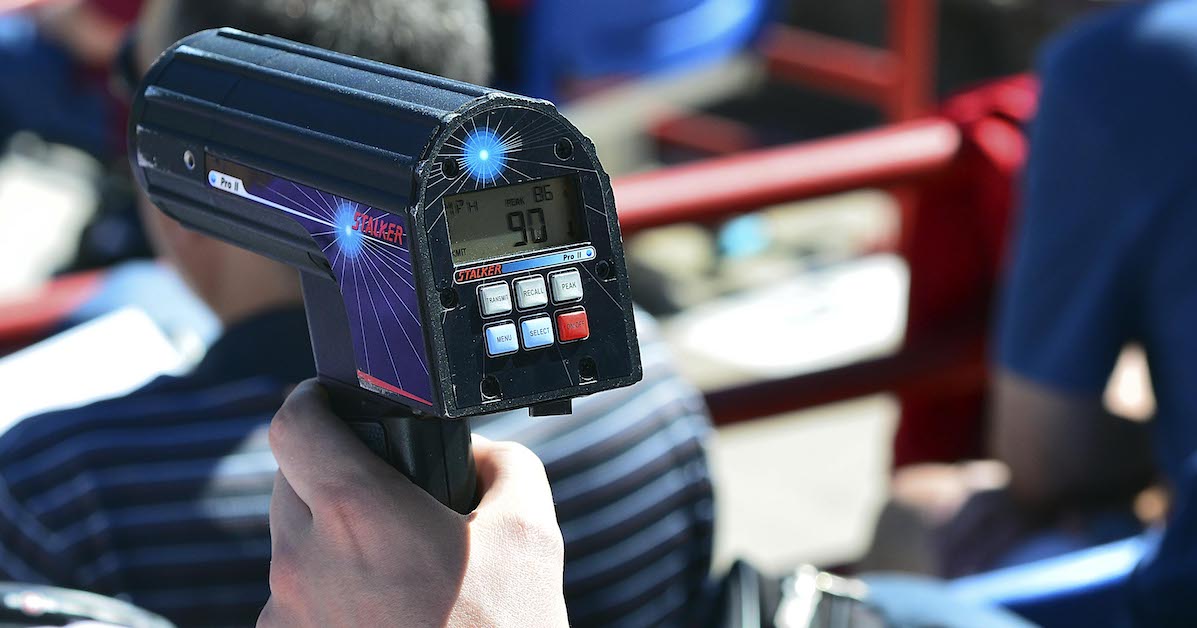
Welcome to another edition of Prospects Week! It’s like Shark Week except with fewer severed limbs, better editing, and a mandatory mention of Hunter Harvey. It’s been a while since we’ve done a thorough-going procedural refresher before getting into the meat of the week, a rundown of what it is we’re doing, why we’re doing it, and how we came to do it this way. For those of you who have been following prospect coverage at FanGraphs for a while, you’ve likely read and/or listened to versions of this before. There have been no significant changes to our process, so for you, the word pile below should mostly serve as a review. For those new to this process, however, welcome! I’m glad you’re here and sorry this is so meticulous.
Let’s start by talking about distributions, the 20-80 scale, and Future Value. Obviously the point of prospect evaluation is to gauge whether a player has the potential to play major league baseball. If the answer to that query is, “yes,” then it’s important to specify how good of a big leaguer we’re talking about. While it comes with its own margin for error, Wins Above Replacement is the best public-facing metric we have for evaluating big leaguers over a meaningful sample. As such, it’s useful for us to try to map our prospect predictions to that metric since it gives us a pretty granular way of distinguishing players from one another. We all know that both Mike Trout and Bryce Harper are very good, but WAR helps us to more precisely understand just how good, and shows us the daylight that exists between players all over the talent and performance spectrum.
Baseball talent is normally distributed across the population, with Trout existing at the far right tail of the continuum, and you and I somewhere toward the far left. When we zoom in and focus solely on the big league portion of the population, the bell curve shape still holds, with Trout on the far right, low-impact players with long careers grouped toward the middle, and career “cup of coffee” types on the far left. This is where the 20-80 scale comes in. This scale has been around baseball (and various scientific fields) forever, and it’s used as a quick means of communicating where a player belongs along the continuum. Using this scale, a “50” is average, and each increment of 10 on either side of 50 represents a standard deviation away from average. The scale doesn’t go from 0-to-100 because the overwhelming majority of data exists within three standard deviations on either side of average, with data existing outside those deviations considered noise. If I was going to graph the height of human men, Joel Embiid and Yao Ming would both be 80s even though Yao has five or six inches on Embiid; seven-footers are three standard deviations away from the 6-foot average, making Yao and others his height noise.
If we map WAR production for hitters to the 20-80 scale, it looks like this:
Scouting Scale | Role | WAR |
---|---|---|
20 | Org guy | — |
30 | Fringe MLB/Triple-A depth | <-0.1 |
40 | Bench player | 0.0 to 0.7 |
45 | Low-end regular/platoon | 0.8 to 1.5 |
50 | Average everyday player | 1.6 to 2.4 |
55 | Above-average regular | 2.5 to 3.3 |
60 | All-Star | 3.4 to 4.9 |
70 | Top 10 overall | 5.0 to 7.0 |
80 | Top 5 overall | > 7.0 |
Don’t let the decimals fool you into thinking this is perfectly exact. These WAR bands are based on actual big league standard deviations, but the lines between the buckets aren’t as stark as the chart makes it seem. The takeaway here is that grades toward the upper end of the curve are rare. In a normal distribution, only about 2% of the population exists two or more standard deviations above the average, which means that in a big-league player population of about 800 guys, only about 10-15 would be “70s” or “80s.”
The problem is that WAR can’t exist in amateur or minor league baseball (for reasons I hope are obvious), which leaves us having to anticipate big league WAR production somehow. To do this, we use current/career big leaguers as a gauge. We try to assess their skills and tools using a combination of visual scouting and relevant data across the same 20-80 scale, then see what kind of player that results in, while keeping in mind both that there will be year-to-year variation and that players continue to develop at the big league level.
Let’s use Javier Báez as an example. Báez has elite bat speed. Visual and data-driven means of evaluating his power put it in the 70-grade area now, though that wasn’t true when he first debuted at age 21. His max-effort swing and aggressive approach can impact the frequency and quality of his contact, and cause him to run hot and cold throughout a season (or for whole seasons at a time). Báez’s career and WAR output provide a template for us to evaluate the prospect version of Bo Bichette. You could MadLib Bo’s name into this entire paragraph and it would still work since he and Báez have very similar offensive talents. That means we can use Báez’s WAR output as a jumping-off point to predict Bichette’s. Bo isn’t as good a defender as Javy, and he doesn’t strikeout quite as much, but they’re similar enough to use one as a foundational heuristic for evaluating the other on the WAR scale before making more subtle adjustments.
Báez’s peak year (5.4 WAR in 2018) is a 70 on the scale, but he hasn’t been that type of player every year, with many seasons in the 2.5-3.0 WAR range. It would be incorrect to call Báez or someone like Carlos Gómez a “70” since there are players who produce 5-ish WAR every year, while Báez and Gómez merely peaked there for a little while. It makes more sense, then, to step back and use the average of a larger, multi-year sample to more accurately understand their true ability and impact. How many years should that sample be and why? That brings us to Future Value, which maps the 20-80 scale to WAR across a player’s six pre-free agency seasons.
Why the six-year bound? Well as our Báez example shows, a multi-year projection is likely more useful than a single-season peak. But without some kind of upper bound, we’re essentially projecting career WAR. That’s tricky, because there are guys like Joakim Soria and Nelson Cruz who end up playing forever. It’s important to have a statistical and cultural appreciation for players like that, but when we’re talking about guys in their teens or early-20s, it’s foolish to try to predict which of them will play as long as a Soria or a Cruz, especially when players who stick around that long tend to shuttle from team to team in free agency. Conversely, when teams are assessing a 28-year-old free agent, they aren’t asking themselves, “What was this guy like as a 21-year-old prospect?” They care about more recent assessments. So when we try to predict the future of prospects at FanGraphs, we are trying to predict the immediate future, the window between when they debut and when they can enjoy a free(ish) market for their abilities.
Let’s return to Báez. In the six years leading up to his free agency, he generated 17.5 WAR, or just shy of 3 average annual WAR. That means that if you were to tell me that a prospect was going to have a career exactly like Báez’s, they would be 60 FV. Things like injury and service time manipulation may impact how much playing time a player has within a given year, which may in turn cause us to prorate performance within a given season to adjust our understanding of a player more precisely, but generally the Future Value grade we put on a player is dictated by what we think their average annual WAR output (mapped to the 20-80 scale) will be during their pre-free agency years of team control. You should be able to take any big leaguer with a career long enough to reach free agency and calculate what an all-knowing prospect writer would have anticipated their FV to be. Mark Reynolds accumulated 9.2 WAR before free agency, or 1.5 average annual WAR, making him a 45 FV. César Hernández? About 2.25 average annual WAR, good for a 50 FV.
A 50 FV indicates that we think a player will be an average everyday big leaguer at their given position. Grades above 50 tell you more precisely how good of an everyday big leaguer we think the prospect will be (recall the Trout/Harper comparison from earlier), while grades below 50 tend to describe role players and everyday players closer to the bottom of the scale. The sample size for role players tends to be smaller, and since WAR is a cumulative stat that is impacted by playing time, the grades for these players map to WAR a little less precisely. It is very hard to be a 50 at first base, since there are so many sluggers at that position. Take Jesús Aguilar, for example. He has made an All-Star team and had one 3-WAR season, but the offensive bar at first base is so high that he’s more often hovered around the 1-WAR mark and has accumulated 5.2 WAR since debuting in 2014. That’s a 40. It is not disrespectful to call Aguilar (or any prospect) a 40, it’s just a contextualization of their place on the baseball talent continuum. Let’s take a look at our WAR/FV chart again, except with role descriptions (and some platonic ideals of those roles) included:
FV | Role | Recent/Current Example |
---|---|---|
30 | Org guy | Darick Hall |
35+ | One-tool bench guy, limited utility | Terrance Gore |
40 | RHH corner role, 5th OF, light-hitting utility, low-end 1B regular, backup C | Jordan Luplow, Guillermo Heredia, Sergio Alcántara, Christian Walker |
40+ | 40 role w/ premium tool or two | Adam Duvall, Edwin Ríos, Adam Engel, Yandy Díaz |
45 | LHH platoon, luxury utility types, low-end MIF/CF regulars | Freddy Galvis, Seth Smith, Matt Joyce, Jackie Bradley Jr. |
What’s with the pluses? For near-ready players, they correspond to the role the chart denotes. For younger players, they’re a way of indicating we think a prospect has a chance to break out and move way up the scale. Would you trade a big-league ready 45 or 50 FV player for the highest-bonus prospect in a given international class? If you made that deal every time, your results would be mixed. You’d swap some good big leaguers for prospects who become great ones, but you’d also swap some good big leaguers for prospects who don’t make it at all. Whether you think it’s smart to make that trade might depend on where your team is on the competitive spectrum, and if that’s the case, it’s helpful in deciding what to do if the players in question have a similar FV, even if one definitely has a higher ceiling than the other. Often, you can make context-based preferential arguments for lots of different players within the same FV tier and still be correct, which we hope readers, players’ parents on social media, and, frankly, other media members will take to heart so they can stop focusing so much on a player’s ordinal rank, and instead concentrate on the evaluation. Especially as it concerns younger players, the “+” tends to indicate that we think the player will have a higher FV in the future, meaning there’s something about them indicative of continued development.
There’s more nuance that abuts this concept, as time and risk are baked into Future Value as well. If I have two identically-projected players and one is at Triple-A and the other is in high school, the one in Triple-A will always be ranked higher than the prepster and probably have a higher FV. If a player is injury-prone or has some other characteristic that increases their perceived risk of busting in our eyes or those of our scouting sources, we’ll tend to dilute the player’s FV. Projecting these guys can be like projecting the path of a hurricane: the farther away you get from the hurricane’s current location, the wider the error bars for its path. We tend to describe this as “variance,” which applies to the year-to-year performance vacillation of big leaguers (Báez would be high variance 60, while Hernández would be low variance 50), as well as being a descriptor of the volatility of younger prospects. Variance can also be a good thing: Tony Sanchez was a low-variance draft prospect, while Trout was a high-variance one. There’s value in the unknown, since what’s unknown may turn out to be positive.
This is especially true for young pitchers, whose elbows play Russian Roulette once a week for about four or five years between when they’re drafted and when they typically establish themselves in a big league role. Pitcher roles have shifted considerably over the last half decade and so has the way we think about them at the site. Because of injury and attrition, teams tend to need many more pitchers than appear on their Opening Day roster to get through a season, so we tend to have lots of pitchers occupying the bottom range of our prospect lists because the industry need for spot starters and up/down relief types is so significant. The pitcher who has a long career as the first or second starter called up from Triple-A in case of injury — let’s say Tommy Milone — is valuable enough to be on a prospect list. On the flip side, the very top of our prospect lists, including our overall Top 100, tends to be dense with hitters. Alex Reyes, A.J. Puk, Forrest Whitley, Yadier Álvarez, MacKenzie Gore — we’ve put our hand on the stove too many times not to make an adjustment in this area. Proximity to the big leagues is also an influential variable for the way we line up pitchers, especially starters. Meanwhile, relievers (who have become more important) are punished by any WAR-based evaluation since they tend to throw fewer innings. We’ve made adjustments to the way we FV relievers to be a little more subjective than just mapping to WAR output. This is one of the benefits of filtering WAR for starters and relievers through FV rather than using WAR itself, as it allows us to make manual adjustments like this. Here is pitcher WAR mapped to the 20-80 scale, along with reliever roles by FV (note that the below tables also make use of the “+” designation):
Scouting Scale | Role | WAR |
---|---|---|
20 | Org guy | – |
35/35+ | Depth/spot starters, typically SP6 through SP8-10 in a given org | < -0.1 |
40 | Fifth starters who eat innings, FIP typically close to 5.00 | 0.0 to 0.9 |
45 | No. 4/5 starters, approx 4.20 FIP, on the fringe of playoff rotation | 1.0 to 1.7 |
50 | No. 4 starters, approx 4.00 FIP, sometimes worse w/lots of IP | 1.8 to 2.5 |
55 | “Mid-rotation” starters, lock for playoff rotation. Approx 3.70 FIP along w/about 160 IP | 2.6 to 3.4 |
60 | All-Star candidates, 3.30 FIP, volume approaching 200 innings | 3.5 to 4.9 |
70 | Top-of-the-rotation types, FIP under 3.00, about 200 IP | 5.0 to 7.0 |
80 | No. 1s. Top 1-3 arms in baseball. ‘Ace’ if they do it several years in a row. | >7.0 |
FV | Role | Recent/Current Example |
---|---|---|
30 | Org guy | C.J. Carter |
35+ | Up/down RP, optioned often | Cole Uvila |
40 | Single-inning middle reliever | Caleb Thielbar |
40+ | Multi-inning reliever, once-through-the-order types | Ryan Yarbrough |
45 | Traditional set-up type, high-leverage guys start here | Alex Colome |
45+ | Likely late-inning RPs with a shot to start | Ryne Nelson |
50 | No-doubt closers | James Karinchak |
That’s a pretty sizable chunk of process-related primer to reference in the near future. There is more of it in this piece from last fall, which details some of how we deal with the problem of putting coherent tool grades on a couple thousand prospects at any given time, and more of it in the Board Tutorial that was republished today, and a tome of it here. I’ll also note that the other prospect publications do not go about prospecting in exactly the same way we do, even though they often also make use of the 20-80 scale. You should be sure to refer to the explanations they provide for their methodology when you engage with them around their work rather than assuming it mimics ours.
Eric Longenhagen is from Catasauqua, PA and currently lives in Tempe, AZ. He spent four years working for the Phillies Triple-A affiliate, two with Baseball Info Solutions and two contributing to prospect coverage at ESPN.com. Previous work can also be found at Sports On Earth, CrashburnAlley and Prospect Insider.
My favorite week <3.
Appreciative of all the work you put into this!