The Spiciest Meatballs of 2024
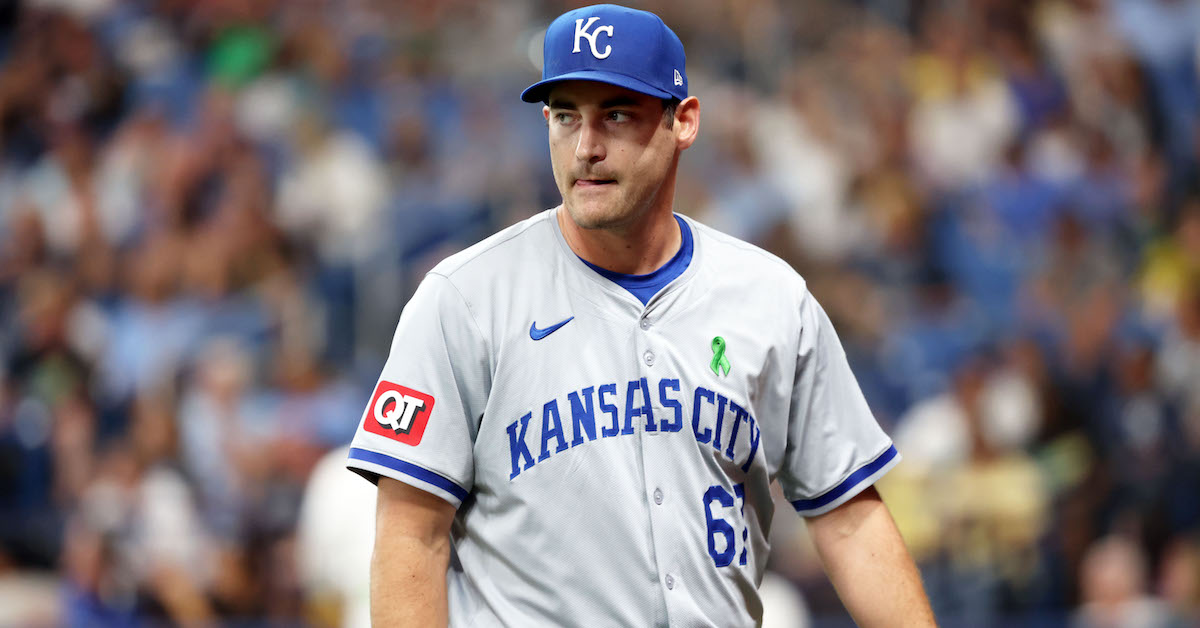
Last week, I cracked the PitchingBot black box open a tiny bit and asked it to show me the worst pitches of the year. It was for fun, mostly; I think there are some interesting data in there, but the main thing I learned was that the worst pitches are non-competitive balls. That’s always a tough concept to grasp, because the ones that stick with us are the hanging sliders and no-ride fastballs right down the pipe, the kind of pitch that we see and go, “Oh I could hit a home run on that.” Like this one:
That’s the worst pitch in baseball this year by one specific metric: the likelihood that PitchingBot assigns it of turning into a home run. I’ll show you some more of them in a moment, but first I thought I’d lay out how I did this so you can get a sense of how the model is reaching its conclusions.
Cameron Grove, the creator of PitchingBot, wrote about this idea back before he started working for the Guardians, and he was kind enough to nudge me in the right direction when it came to looking at pitches not just for the “worst,” but the ones that are the most crushable.
The model might spit out one run value for each pitch, but that’s not how it works internally. As Grove described in the primer that was published when we added PitchingBot grades to our site, the model is comprised of many sub-models that try to predict the likelihood of different discrete events. The grade of any single pitch follows this delightful flowchart:
We aren’t interested in most of these boxes, so first we need to calculate the odds that a ball ends up in play so that it can become a home run. The “no swing model” section, with its chances of HBPs and balls, contains a ton of drivers of truly awful pitches. The swing model has some outputs we don’t care about – swinging strikes and foul balls aren’t what we want. We’re only looking for the ball-in-play model, so the first thing I did was go through each pitch to find the likelihood of a swing that results in non-foul contact.
Let’s use the confrontation between Seth Lugo and Randy Arozarena from the beginning of this piece as an example. The model looks at count when coming up with the probability of a swing, as it should: Batters behave differently in 3-0 counts than they do when they’re behind 0-2. The model assigned an 82.8% chance of a swing on this particular pitch. On 3-1, batters are often looking for something right down the middle, and that means they often swing when they get it.
Next, the model assigns chances of a whiff (given a swing) and chances of a foul ball (given contact). On this pitch, the model didn’t give much probability weight to a swinging strike; it predicted a contact rate of 87.7%. It also predicted a foul ball rate of 45%. The point of this article isn’t to figure out those values – that’s what the model is for – but just for a sanity check, fastballs right down the middle in 2024 have resulted in an 87.3% contact rate and a 41.6% foul ball rate. Seems like that’s right in line with expectations, then.
Multiply all of these together, swing rate times contact rate times one, and then subtract the foul ball rate, and you’ll get the model-predicted odds of a ball in play. That works out to a 39.9% chance of a ball in play on this particular pitch. Given that Arozarena didn’t swing, we know the actual outcome wasn’t a ball in play, but the model’s working in generalities here. Pipe shot fastballs in 3-1 counts result in balls in play fairly often.
From there, the model estimates the probability of a number of different types of batted balls. Roughly speaking, it breaks out five different speed ranges (<90, 90-95, 95-100, 100-105, 105+) across grounders, line drives, and fly balls. That makes 15 buckets; the model assigns a chance that successful fair contact will result in a ball in each bucket, then assigns an average run value for balls hit with that combination of speed and angle.
We’re diverging from the main model here, though. That’s all well and good when you’re wondering what kind of production a given swing will produce, but I don’t care about average production for my purposes here. I care about how likely a pitch is to produce a home run. So I threw out all the run values, and replaced them with home run probabilities. All the groundball buckets have a 0% chance of producing a homer. Line drives hit between 95 and 100 mph have resulted in 16 homers out of 3,922 batted balls, a 4.1% homer-per-BIP rate. Fly balls hit 105 mph or harder have turned into home runs 80.3% of the time — 1,586 homers out of 1,976 batted balls. I did this for all the buckets to give me a home run probability for each batted ball type. Then I multiplied the model-predicted likelihood of each bucket by the home run probability of that bucket and summed them all up to get the chance of a ball in play turning into a homer.
Sticking with the Lugo pitch, when hitters put a fastball like that into play, the model predicts mostly fly balls, 58.8% of the time. Plenty of those are weak fly balls, of course. There’s no pitch you could throw that gets hammered every single time. But if you sum it all up, across all the buckets and the likelihood of homers in each, you get a 16.6% chance that a ball in play, against this pitch, would result in a home run.
That might not sound like a huge number, but consider this. Aaron Judge is setting baseball ablaze with his colossal power right now. Over the last three years, he’s been outrageously good. He’s hitting .304/.431/.670, good for a 202 wRC+. In that stretch of time, he’s converted 15.2% of his batted balls into homers.
That meatball Lugo threw to Arozarena? It turns the average major league hitter into Aaron Judge – if they swing. I can kind of believe it. That pitch was about as smashable as they come: 92 mph, a full two mph below league average. Dead middle, a horrible location. Unexceptional movement. It’s not that it always turns into a home run – 16.6% isn’t even a quarter of the time, and pitchers get away with these frequently – but if Arozarena had launched that ball into orbit, no one would’ve been surprised.
Take the 39.9% chance of a ball in play on this pitch from up above, multiply it by the 16.6% chance of a homer on that ball in play, and you get a 6.3% chance of a home run. That’s wildly bad. There have been 517,841 pitches this year, and 3,991 home runs. That works out to a rate just under 0.8%. Lugo’s pitch was eight times more likely than the average pitch to turn into a homer. Woof. Even pitches in the strike zone only get hit for homers 1.4% of the time.
I lied, very slightly, up above. I said that the fastball to Arozarena was the meatball-iest meatball of the year, that the only sticky stuff on that pitch was a nice marinara sauce. But the model found three pitches that were even more likely to result in home runs. Here’s the overall winner, with a 7.5% chance of leaving the yard:
That’s a brief glimpse, I know. This was the only video feed for the game, and the camera cut in midway through the pitch. But it was a 56.4 mph “fastball” from Barry Bonds cosplayer Tyler Fitzgerald, who was wearing the last inning of a 17-1 shellacking to save the Giants’ bullpen. That’s a Home Run Derby fastball; Fitzgerald was up there throwing batting practice. In fact, the top three most homerable pitches were all thrown by position players, so I excluded them.
With those criteria in mind, I think that Lugo’s fastball was the most crushable pitch thrown this season — and Arozarena didn’t even swing! But second on the list, at 6.1%? That one led to some fireworks, and also a very sad Drew Smyly:
It’s almost uncanny how similar those pitches are, just unexceptional fastballs in the worst imaginable location. That’s kind of the point. The model knows what it’s talking about when it comes to homer-prone pitches. Let’s check out another one. Tyler Anderson said, Here, hit this (6.1% homer chance):
On another night, Jorge Polanco might have crushed that ball. But he was late swinging and managed to break his bat on a pitch down the middle, and with one of his slowest swings of the year to boot. Sometimes they lob one in there and you just aren’t ready for it. Sometimes you’re just a fraction of a second early or late. It happens.
One notable fact about the pitches most likely to get hit for home runs: They’re pretty much all fastballs. That’s not to say that bad breaking balls aren’t likely to get smashed. Here’s a bad cutter, with a 4.7% chance of turning into a homer:
And a bad slider, 4.5%:
I have two points in showing you all of these pitches. First, they’re fun to see. A good portion of major league hitters’ daily routine involves having to make tough decisions about pitches where there are no good options. A nasty slider shaving off the low-and-away corner? Good luck, buddy. High, riding fastballs? Hope you like swinging and missing. But sometimes they get these cookies instead, and even then, most of them don’t leave the yard. Hitting is so hard!
Another reason I’m showing you these is to demonstrate the face validity of the model. It’s not just spouting gibberish. When it says a pitch is extremely crushable, it’s considering shape, speed, location, and count. The answers it’s coming up with are eminently believable. I looked at every single one of the pitches in here and thought, “Yep, meatball.”
If you believe in the rough contours of the metric, I can do things with it. Here, for example, are the pitchers (minimum 750 pitches graded by PitchingBot in 2024) who throw the most meatballs. I followed Grove’s lead from his earlier article and defined a meatball as any pitch with a 3% chance or higher of turning into a home run:
Pitcher | Meatballs | Meatball Rate | HR/9 | GB/FB |
---|---|---|---|---|
Joey Estes | 72 | 5.6% | 1.20 | 0.43 |
Tobias Myers | 70 | 5.3% | 1.25 | 0.94 |
Ben Lively | 85 | 5.1% | 1.66 | 0.86 |
Ross Stripling | 54 | 4.8% | 0.87 | 1.02 |
Bailey Falter | 67 | 4.8% | 1.15 | 0.81 |
Simeon Woods Richardson | 79 | 4.6% | 0.97 | 0.89 |
Slade Cecconi | 51 | 4.6% | 1.72 | 0.68 |
Ronel Blanco | 90 | 4.6% | 1.34 | 0.88 |
Matt Waldron | 60 | 4.6% | 1.09 | 0.85 |
Chris Flexen | 92 | 4.5% | 1.45 | 0.73 |
In aggregate, these guys are fly ball pitchers who give up homers. It’s probably no accident that there are two A’s on the list; the Coliseum is cavernous and turns plenty of those mistakes into long fly outs. If Rockies and Reds pitchers served up this many meatballs, they wouldn’t be around for long; you can’t survive in those parks if you offer up smashable pitches with any regularity.
On the flip side of the ledger: Tyler Rogers, Justin Lawrence, Kevin Kelly, and Justin Martinez each have recorded 750 or more pitches without throwing a single meatball. Logan Webb has thrown 2,439 pitches this year, the most in baseball, and only five of them were meatballs. The next-stingiest full-time starter is Cristopher Sánchez, and he throws meatballs at roughly double Webb’s rate. It’s hard to avoid throwing absolutely awful pitches, but for the pitchers who can do it consistently, it clearly pays off.
With this neat little corner case use of PitchingBot now discovered, I’m planning on going a few ways with this research. First, I’m going to see if I can find any promising signal in these data that I can use to analyze pitchers better. I’m not particularly confident that I’ll find anything here, to be honest. Webb good: noted. Estes bad: sure, I suppose. But fly ball pitchers throw more of these absolute cookies, and they generally throw more pitches that batters swing through as well, so there’s some anti-correlation to deal with. It’s a promising area of research, but it’s hard to find new signals that other pitching statistics don’t already cover.
Second, I’m going to mine it for content, obviously. “Did you see that absolute meatball that Pitcher X threw?” is a frequent question in my chats. “How hittable did Pitcher Y look this weekend?” is another one. I generally don’t answer those questions unless I watched the game – how else would I know? But thanks to the magic of carefully constructed algorithms, now I can have an answer, and it’s easy to find the most crushable pitches of the week, or the pitcher who threw the most meatballs, anything like that. If you’re wondering which pitchers are doing their part to increase runs via home runs, I think it just got a lot easier to find the answer.
For now, though, I’ll just leave you with this: No matter how bad a pitch is, no matter what count it’s thrown in, and no matter who is batting, it’s never likely to become a home run. That doesn’t make it a good pitch. It makes it an extremely terrible pitch, in fact. But even these juicy pitches frequently end in tears for the hitter. That’s just baseball: Most of the time, batters lose. But the odds of success aren’t always the same, and PitchingBot does a great job of showing when they’re at their most tilted.
Ben is a writer at FanGraphs. He can be found on Bluesky @benclemens.
If you’re into the whole brevity thing, might I suggest Expected Destruction?
XD for short, obviously