A New Way of Looking at Depth
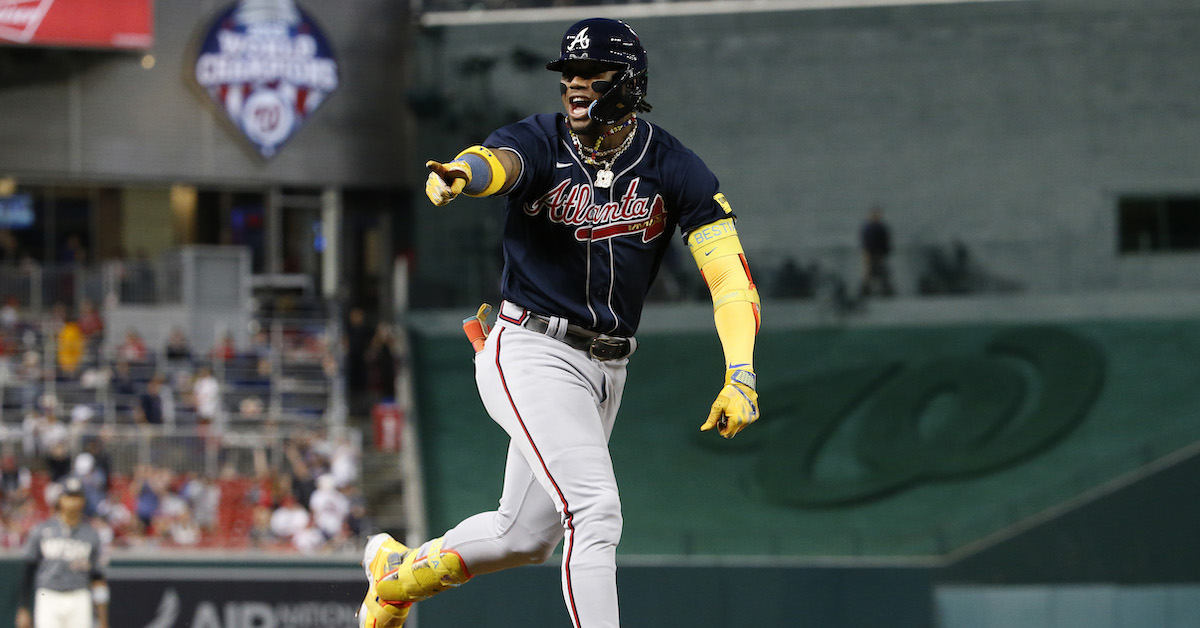
One of the great perks of working at FanGraphs is that I get to discuss baseball with my equally obsessed coworkers. Obviously, this is the kind of job you don’t get into unless you love the sport. A lot of the time, that means we just end up nerding out over how much we enjoy some minor but cool thing, or perhaps discussing our favorite of the game’s idiosyncrasies. Sometimes, though, we come up with new ideas together, or one person’s passing fancy turns into another person’s brainstorm, and before you know it, something nifty and novel is happening.
That’s why I’m writing this article today. At the December Winter Meetings, a subset of us sat down for our annual let’s-talk-about-fun-baseball-problems technical meeting. David Appelman and Sean Dolinar ran things. Folks like Jeff Zimmermann and Dan Szymborski popped in at various points. Jason Martinez and Keaton Arneson had big plans for how to improve the site’s functionality. Those guys are great at building models, running websites — advancing the state of how FanGraphs (and ZiPS) works, basically. I like to make jokes and write articles about bunts, so as far as I can tell, I got invited because I’m good at coming up with bad but interesting ideas.
That said, this year one thing was on a lot of our minds: depth. I’ve written a lot about how well our playoff odds reflect reality. They’re pretty good! But there’s always been an obvious problem with them. They use static rosters, which means they don’t account for the fact that some teams are more vulnerable to injury or underperformance than others.
The reason things work this way is straightforward. To run our playoff odds model, we begin by constructing an estimate of each team’s strength. We come up with that estimate from the bottom up. First, we project the playing time that each player receives — or rather, we use Jason Martinez’s playing time estimates, which feed our depth charts and RosterResource. Then we estimate how those players will perform using a blend of ZiPS and Steamer projections. We add those all up, sprinkle in a little BaseRuns magic, and voila! We have estimates of how many runs a team will score and allow per game, which you can convert into expected winning percentages.
As I’ve written in several similar explainers before, the best part of this model is its simplicity. It runs more or less continuously throughout the day; every time a new game ends, our odds change to reflect the result. There’s not much chance of some kind of weird model outcome we can’t explain; we know who’s getting the playing time and how good we expect them to be, so there’s just nowhere for it to break (sorry, David, I’m sure I jinxed something by saying this).
The big weakness of this plan? Let’s take a look at the Braves’ depth chart for a simple example. Ronald Acuña Jr. is projected for 679 plate appearances. In every one of the 20,000 simulations we run each time the model updates, we pencil Acuña in for exactly 679 plate appearances. But that’s not how things have gone. Last year, he had 735 plate appearances. In 2021, he had only 360 thanks to injury. Obviously, the Braves would be a good deal worse if Acuña were to miss time, but because of the way we run things, we’re not capturing that possibility (though should some misfortune befall Acuña, the depth charts and the playoff odds would reflect it in a hurry).
We’ve known about this limitation of the model construction for quite a while, and from time to time we’ve tried to brainstorm solutions. Some very helpful readers of the site have wasted hours trying to explain fancy math to me. My friends have given me suggestions. We’ve talked about various options internally. But this winter, Appelman figured something out, and I’m delighted to share the rough contours of it with you today.
It started with an idle thought: What if you just removed random players from each roster? You could rebuild the team without them, figure out the difference in team quality, and run the odds with that new, post-removal estimate of team strength as a proxy for the chance of injury. Roll enough dice for each team on each run, and maybe random removal could do a good job approximating the vagaries of health.
As it turns out, that’s easier said than done. It’s computationally intensive, for one thing. We’re also not set up to rebuild a roster on the fly. But this idea of subtracting individual players and working out team strength from there struck a chord with all of us, and as the winter went on, Appelman kept sending me new iterations of his approach to the problem.
At one point, we wanted to estimate the strength of a team’s starters, its listed second string, and its listed third string. Then we could just interpolate between the three, or perhaps randomly weight each group, to get an estimate of team winning percentage that took into account the likelihood of its backups getting significant playing time. That worked alright most of the time, but it’s actually hard to define a team’s “second string.” I specifically remember looking at a Cardinals depth chart at one point this winter. The second string, if you just took the player projected for the second-most plate appearances at each spot in the field, included three Brendan Donovan’s and three Tommy Edman’s. Right, well, that probably wouldn’t work then.
After plenty of trial and error, though, Appelman and team have come up with a really cool solution. It’s a variation on the original idea, in fact. Rather than thinking of it as a playoff odds tool, the idea is to create a grid that shows how each team’s expected winning percentage changes as we remove the top players from the roster. First, we calculate the full-strength numbers against neutral opposition, which are what you can see on our projected standings page. Then we lop off the player with the most projected WAR and increase the plate appearances (or innings pitched) of the guys below that player on the depth chart to refill the team’s playing time.
Let’s use Acuña as an example again. He’s projected to be the Braves’ best player, of course. Our process gets rid of his entire season and replaces it with plate appearances for other players on Atlanta’s roster. Jarred Kelenic is second on the depth chart, but he can’t get all 679 of Acuña’s plate appearances; he’s already projected for 525 plate appearances as the team’s everyday left fielder. Even Cal Ripken Jr. couldn’t top 1,200 plate appearances in a year, so Appelman’s algorithm is a bit more complicated than that.
The process first adds a missing player’s playing time to his top backup, but then there’s a check: If someone has more than a full season’s playing time (700 non-catcher PA, 640 catcher PA, or 200-ish innings pitched for a starter), the algorithm caps them at 100% playing time. It subtracts those “extra” projected PAs/IP proportionally from each position. In Kelenic’s case, that means he’d lose mostly right and left field playing time, with a tiny bit of center field to boot. Then those freed-up plate appearances get kicked down the line – at each of the three outfield spots, Forrest Wall is the next man up after Kelenic, and he’s only projected for 98 plate appearances right now, so he soaks up the entire remainder of the playing time.
As you can imagine, that makes the Braves a lot worse. Specifically, when we recalculate their expected winning percentage without Acuña, they fall from a .598 team to a .567 squad. That 31-point decline is the biggest that any team in baseball would suffer if their best player got completely replaced by backups. That makes sense – Acuña has the best projection not just on the Braves but in all of baseball, and Atlanta’s options behind him are lackluster. For comparison, the Cubs project to suffer the smallest decline in performance. We have Nico Hoerner down as their best player, and we like a lot of the options behind him; Nick Madrigal, Michael Busch, and Patrick Wisdom can absorb a lot of infield innings between them with admirable aggregate projections.
From there, our new process just keeps going. Next, we lop off the top two players on each team. We’ll stick with Atlanta; this time, Acuña and Spencer Strider are both out. In the case of a pitcher, we hand out playing time in terms of starts, with no player getting more than 20% of the starts. So every other starter picks up some of the slack, with Bryce Elder and Huascar Ynoa seeing the greatest increases. It’s the same process behind the scenes, though. First, Max Fried gets all of Strider’s 180 innings, but then he has way too many, so we push his excess innings down the chain to Charlie Morton. That results in Morton having way too many, so they get pushed down the line, and so on. There’s only one exception: players who are currently injured are specifically excluded from having their playing time increased, which prevents a starter currently ticketed to return from TJ in the second half of the year from soaking up unrealistic innings.
We’ve run this process pretty far down the roster – until we’ve eliminated each team’s top 10 projected performers, to be precise. These depth charts look pretty dire; remove any team’s best 10 players, and things get a lot worse! To stick with the Braves as an example, try to imagine them without Acuña, Austin Riley, Michael Harris II, Matt Olson, Ozzie Albies, Sean Murphy, Strider, Fried, Morton, and Chris Sale. Our method thinks that they’d be a .452 team (against league average opposition) if those 10 missed the entire season.
We have to take a few shortcuts to handle cuts of that magnitude, because there aren’t always enough players listed at each position to make it work, and some very deep backups end up with over-allocated playing time, but at the point where you’re wondering who should pick up J.P. Martínez’s projected playing time from among the Braves’ non-listed options, the overall effect on their winning percentage is quite small. That means that there are some weird discrepancies here and there when you get all the way down the playing time ladder, but they’re tiny in magnitude and as best as I can tell, not worth worrying about. We think the Braves improve from a .452004 team to a .452197 team, for example, when we remove their 10th-best player and backfill all that playing time, but that just comes down to which replacement-level player we’re handing that playing time to. A quick clarifying note here: Since the model can only work with our existing depth charts, only players projected for at least one plate appearance or batter faced enter into our calculations. That doesn’t perfectly match reality, but this far down the depth chart, most teams are picking between a variety of replacement-level players anyway, so we don’t think the model loses much from that limitation.
Okay, you’ve stuck around for all the rambling, so let’s get down to brass tacks. You’re reading this article because you want to know who does well by this method. Here’s the giant raw grid of team winning percentage as we lop players off of each team’s roster:
Team | 0 | 1 | 2 | 3 | 4 | 5 | 6 | 7 | 8 | 9 | 10 |
---|---|---|---|---|---|---|---|---|---|---|---|
Braves | .598 | .567 | .551 | .532 | .518 | .502 | .485 | .473 | .462 | .452 | .452 |
Dodgers | .573 | .556 | .538 | .525 | .506 | .500 | .494 | .484 | .480 | .462 | .455 |
Astros | .560 | .537 | .517 | .495 | .481 | .469 | .463 | .455 | .449 | .443 | .435 |
Yankees | .549 | .530 | .504 | .494 | .487 | .477 | .471 | .465 | .457 | .450 | .451 |
Rays | .534 | .526 | .518 | .511 | .502 | .495 | .490 | .485 | .488 | .484 | .485 |
Mariners | .528 | .508 | .497 | .477 | .465 | .462 | .452 | .443 | .440 | .434 | .425 |
Orioles | .525 | .513 | .503 | .491 | .484 | .474 | .469 | .466 | .460 | .458 | .453 |
Phillies | .523 | .510 | .493 | .479 | .458 | .450 | .446 | .439 | .424 | .424 | .420 |
Blue Jays | .522 | .515 | .503 | .487 | .476 | .472 | .466 | .463 | .462 | .450 | .452 |
Twins | .521 | .510 | .499 | .491 | .483 | .473 | .466 | .458 | .453 | .451 | .445 |
Diamondbacks | .515 | .503 | .490 | .480 | .467 | .461 | .448 | .447 | .438 | .435 | .434 |
Cardinals | .514 | .506 | .496 | .491 | .487 | .482 | .474 | .476 | .471 | .472 | .472 |
Rangers | .506 | .492 | .482 | .478 | .471 | .459 | .453 | .449 | .443 | .437 | .428 |
Cubs | .501 | .500 | .496 | .490 | .485 | .478 | .466 | .467 | .474 | .466 | .471 |
Red Sox | .501 | .488 | .473 | .474 | .468 | .468 | .463 | .457 | .461 | .460 | .450 |
Padres | .500 | .475 | .462 | .450 | .442 | .436 | .428 | .424 | .416 | .410 | .411 |
Brewers | .497 | .490 | .483 | .473 | .463 | .459 | .449 | .451 | .450 | .450 | .448 |
Mets | .496 | .482 | .474 | .462 | .457 | .444 | .438 | .433 | .428 | .425 | .425 |
Giants | .495 | .483 | .490 | .484 | .481 | .476 | .473 | .472 | .469 | .457 | .452 |
Marlins | .495 | .491 | .485 | .477 | .468 | .459 | .448 | .438 | .430 | .430 | .428 |
Guardians | .493 | .476 | .469 | .463 | .457 | .450 | .439 | .431 | .419 | .416 | .411 |
Tigers | .489 | .479 | .476 | .466 | .458 | .457 | .458 | .456 | .454 | .459 | .456 |
Reds | .488 | .485 | .482 | .477 | .477 | .474 | .471 | .466 | .453 | .451 | .449 |
Angels | .481 | .466 | .455 | .450 | .442 | .433 | .428 | .422 | .420 | .410 | .399 |
Pirates | .477 | .470 | .465 | .460 | .451 | .439 | .441 | .438 | .436 | .438 | .428 |
Royals | .467 | .450 | .441 | .429 | .424 | .425 | .425 | .425 | .426 | .425 | .423 |
Athletics | .443 | .437 | .436 | .426 | .424 | .424 | .421 | .423 | .419 | .417 | .412 |
White Sox | .417 | .402 | .392 | .384 | .375 | .363 | .358 | .353 | .357 | .354 | .360 |
Nationals | .408 | .393 | .386 | .379 | .374 | .372 | .367 | .363 | .360 | .367 | .367 |
Rockies | .394 | .387 | .383 | .381 | .377 | .375 | .374 | .374 | .374 | .365 | .365 |
Yeah, that’s an overwhelming amount of data, and the table is sortable in case you want to play around with it. In terms of broad conclusions, the teams least affected by dropping their 10 best players are largely the ones that are already bad. The Rockies, Athletics, Nationals, and Royals are all in the top 10 of least-affected teams, and they sport four of our five lowest projected winning percentages at full strength. Pity the poor White Sox, who have so little depth that they managed to get quite a bit worse even starting from such a low floor. One quick note on all of these, by the way: In each case, we’re only removing players from the team in question. When we say that the Braves project for a .452 winning percentage with 10 players gone, that’s against an otherwise normal league.
The teams with the most to lose in such a complete roster destruction are basically just the best teams. The Braves see their winning percentage decline the most, with the Astros and Dodgers hot on their heels. Some interesting exceptions to this rule: The Cardinals, Rays, and Cubs have projected full-strength records above .500 but all finish in the top 10 for least-injury-impacted. In other words, they have capable replacements in addition to starters good enough to compete for the playoffs on their own merits. Those are the types of teams that our current odds method tends to underrate. The Brewers and Reds only miss that group because we think they’re just worse than .500 at full strength; in other words, the entire NL Central has pretty excellent depth.
Perhaps a more interesting question is which teams suffer the most from a more realistic number of injuries – say three top stars. The Braves and Astros are head and shoulders above the field here. But make no mistake: Those teams are still really good. Those two might project to suffer the biggest decline in performance due to injury, but we still think they’d be top-five teams in baseball in a hypothetical world where every organization was missing its best three players. Here, you can sort by either change in winning percentage or absolute winning percentage with three players missing to see how even with those big losses, Atlanta’s squad is built to crush:
Team | Full Strength | 3 Missing | Change |
---|---|---|---|
Braves | .598 | .532 | -.066 |
Dodgers | .573 | .525 | -.048 |
Astros | .560 | .495 | -.065 |
Yankees | .549 | .494 | -.055 |
Rays | .534 | .511 | -.023 |
Mariners | .528 | .477 | -.051 |
Orioles | .525 | .491 | -.034 |
Phillies | .523 | .479 | -.044 |
Blue Jays | .522 | .487 | -.034 |
Twins | .521 | .491 | -0.03 |
Diamondbacks | .515 | .480 | -.036 |
Cardinals | .514 | .491 | -.024 |
Rangers | .506 | .478 | -.028 |
Cubs | .501 | .490 | -.012 |
Red Sox | .501 | .474 | -.027 |
Padres | .500 | .450 | -.049 |
Brewers | .497 | .473 | -.024 |
Mets | .496 | .462 | -.034 |
Giants | .495 | .484 | -.011 |
Marlins | .495 | .477 | -.017 |
Guardians | .493 | .463 | -0.03 |
Tigers | .489 | .466 | -.024 |
Reds | .488 | .477 | -.011 |
Angels | .481 | .450 | -.031 |
Pirates | .477 | .460 | -.016 |
Royals | .467 | .429 | -.038 |
Athletics | .443 | .426 | -.016 |
White Sox | .417 | .384 | -.032 |
Nationals | .408 | .379 | -.029 |
Rockies | .394 | .381 | -.013 |
In fact, the Braves project for a .501 winning percentage even if we remove their top five players from the depth chart. In some ways, sure, they’re a thin team. But one way to weather an injury storm is to be so dang good that the rest of your starters can pick you up, and that’s where Atlanta is this year.
When you lop six players off of each team, the Dodgers have the best projected winning percentage. At seven players, the Rays take a tiny edge, and with each remaining gradation, they have the best projection. The Cardinals aren’t far behind by that point. But even with 10 players gone, the Braves have the seventh-best projection in the majors. It’s a completely different way of thinking about depth than I’m used to, but I think it’s a really cool result.
The best part about all of this? With enough massaging and reformatting of the way we do our playoff odds, and with plenty of careful calibration to handle how often to call up each injury scenario for each team, we can eventually integrate this knowledge into our projections. In some potential future, the Braves might be missing their top two players – or some combination of the middle players that affects their winning percentage in a similar way – while the Phillies and Mets have pristine health. How would the division go in that case? That could be one of our runs.
Drawing from each team’s injury table independently does a better job of simulating the many possible outcomes of a season than using a static depth chart. Want to know what the Rays’ vaunted depth is worth? Over a giant batch of simulations, their poor injury draws hurting them less than other teams will give them an advantage. Likewise, I doubt Atlanta’s lack of depth is a huge issue, because the team is just so good to begin with.
I don’t have the answers to that part of the equation today. We – and by we, I mainly mean Appelman – haven’t worked out how to integrate this new way of looking at depth and injury into our existing model. Adding this methodology to our playoff odds will require careful calibration and some reworking of the existing code. But that’s something we’re working on, and I think the results of this project are already plenty cool. It’s all well and good to say that depth matters. Here, now, we can say how much it matters, and for which teams.
One note to pre-empt a question I’m sure you’re wondering about: lopping off a team’s best players in order doesn’t exactly reflect reality. Neither does having those players miss the entire season instead of some portion of it. That’s true. But we’re trying to create an abstraction here, because all models are abstractions of reality in one way or another. We think this is a pretty good one – it’s telling us something useful about team composition that our existing option misses. I think it’s fascinating, and I hope you will as well.
Ben is a writer at FanGraphs. He can be found on Bluesky @benclemens.
What an awesome undertaking.
Excited to see how it evolves.