The 2025 ZiPS Projections Are Imminent!
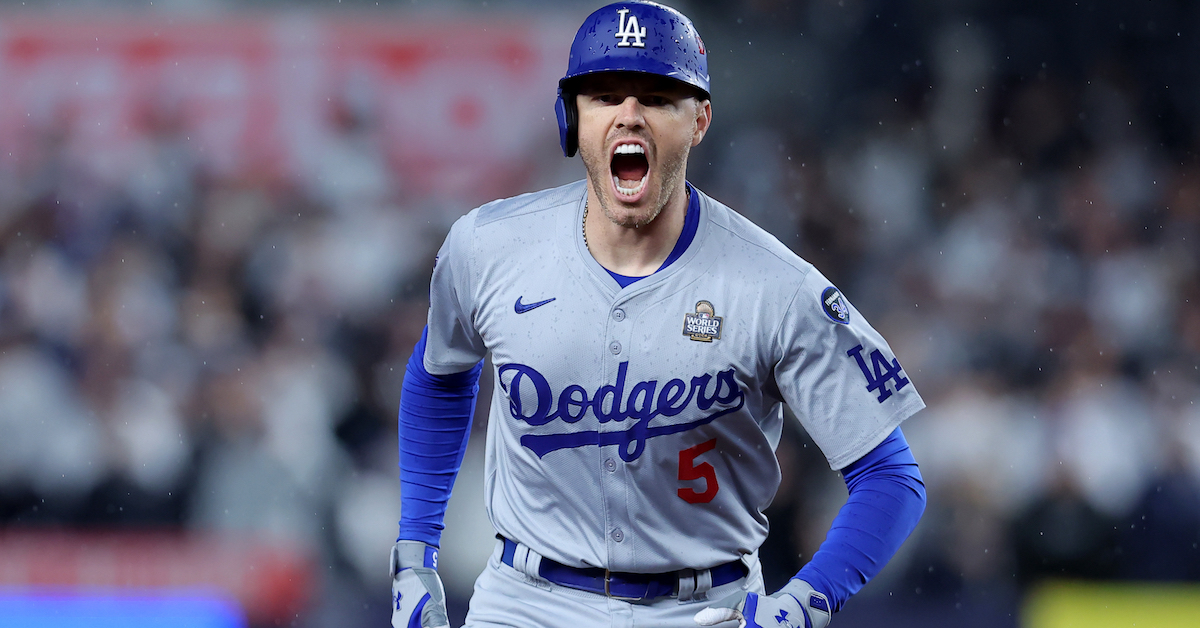
Well, it’s that time of the year again. When the last gasps of summer weather finally die and everybody starts selling pumpkin spice everything, that’s when I make the magical elves living in the oak in my backyard start cranking out the E.L.fWAR cookies. Szymborski shtick, Szymborski shtick, pop culture reference, and now, let’s run down what the ZiPS projections are, how they work, and what they mean. After all, you’re going to be seeing 30 ZiPS team articles over the next two months.
ZiPS is a computer projection system I initially developed in 2002–04. It officially went live for the public in 2005, after it had reached a level of non-craptitude I was content with. The origin of ZiPS is similar to Tom Tango’s Marcel the Monkey, coming from discussions I had in the late 1990s with Chris Dial, one of my best friends (our first interaction involved Chris calling me an expletive!) and a fellow stat nerd. ZiPS quickly evolved from its original iteration as a reasonably simple projection system, and now does a lot more and uses a lot more data than I ever envisioned it would 20 years ago. At its core, however, it’s still doing two primary tasks: estimating what the baseline expectation for a player is at the moment I hit the button, and then estimating where that player may be going using large cohorts of relatively similar players.
So why is ZiPS named ZiPS? At the time, Voros McCracken’s theories on the interaction of pitching, defense, and balls in play were fairly new, and since I wanted to integrate some of his findings, I decided the name of my system would rhyme with DIPS (defense-independent pitching statistics), with his blessing. I didn’t like SIPS, so I went with the next letter in my last name, Z. I originally named my work ZiPs as a nod to CHiPs, one of my favorite shows to watch as a kid. I mis-typed ZiPs as ZiPS when I released the projections publicly, and since my now-colleague Jay Jaffe had already reported on ZiPS for his Futility Infielder blog, I chose to just go with it. I never expected that all of this would be useful to anyone but me; if I had, I would have surely named it in less bizarre fashion.
ZiPS uses multiyear statistics, with more recent seasons weighted more heavily; in the beginning, all the statistics received the same yearly weighting, but eventually, this became more varied based on additional research. And research is a big part of ZiPS. Every year, I run hundreds of studies on various aspects of the system to determine their predictive value and better calibrate the player baselines. What started with the data available in 2002 has expanded considerably. Basic hit, velocity, and pitch data began playing a larger role starting in 2013, while data derived from Statcast has been included in recent years as I’ve gotten a handle on its predictive value and the impact of those numbers on existing models. I believe in cautious, conservative design, so data are only included once I have confidence in their improved accuracy, meaning there are always builds of ZiPS that are still a couple of years away. Additional internal ZiPS tools like zBABIP, zHR, zBB, and zSO are used to better establish baseline expectations for players. These stats work similarly to the various flavors of “x” stats, with the z standing for something I’d wager you’ve already guessed.
How does ZiPS project future production? First, using both recent playing data with adjustments for zStats, and other factors such as park, league, and quality of competition, ZiPS establishes a baseline estimate for every player being projected. To get an idea of where the player is going, the system compares that baseline to the baselines of all other players in its database, also calculated from the best data available for the player in the context of their time. The current ZiPS database consists of about 145,000 baselines for pitchers and about 180,000 for hitters. For hitters, outside of knowing the position played, this is offense only; how good a player is defensively doesn’t yield information on how a player will age at the plate.
Using a whole lot of stats, information on shape, and player characteristics, ZiPS then finds a large cohort that is most similar to the player. I use Mahalanobis distance extensively for this. A few years ago, Brandon G. Nguyen did a wonderful job broadly demonstrating how I do this while he was a computer science/math student at Texas A&M, though the variables used aren’t identical.
As an example, here are the top 50 near-age offensive comparisons for World Series MVP Freddie Freeman right now. The total cohort is much larger than this, but 50 ought to be enough to give you an idea:
Ideally, ZiPS would prefer players to be the same age and play the same position, but since we have about 180,000 baselines, not 180 billion, ZiPS frequently has to settle for players at nearly the same age and position. The exact mix here was determined by extensive testing. The large group of similar players is then used to calculate an ensemble model on the fly for a player’s future career prospects, both good and bad.
One of the tenets of projections that I follow is that no matter what the ZiPS projection says, that’s what the projection is. Even if inserting my opinion would improve a specific projection, I’m philosophically opposed to doing so. ZiPS is most useful when people know that it’s purely data-based, not some unknown mix of data and my opinion. Over the years, I like to think I’ve taken a clever approach to turning more things into data — for example, ZiPS’ use of basic injury information — but some things just aren’t in the model. ZiPS doesn’t know if a pitcher wasn’t allowed to throw his slider coming back from injury, or if a left fielder suffered a family tragedy in July. Those sorts of things are outside a projection system’s purview, even though they can affect on-field performance.
It’s also important to remember that the bottom-line projection is, in layman’s terms, only a midpoint. You don’t expect every player to hit that midpoint; 10% of players are “supposed” to fail to meet their 10th-percentile projection and 10% of players are supposed to pass their 90th-percentile forecast. This point can create a surprising amount of confusion. ZiPS gave .300 batting average projections to two players in 2024: Luis Arraez and Ronald Acuña Jr. But that’s not the same thing as ZiPS thinking there would only be two .300 hitters. On average, ZiPS thought there would be 22 hitters with at least 100 plate appearances to eclipse .300, not two. In the end, there were 15 (ZiPS guessed high on the BA environment for the second straight year).
Another crucial thing to bear in mind is that the basic ZiPS projections are not playing-time predictors; by design, ZiPS has no idea who will actually play in the majors in 2025. Considering this, ZiPS makes its projections only for how players would perform in full-time major league roles. Having ZiPS tell me how someone would hit as a full-time player in the big leagues is a far more interesting use of a projection system than if it were to tell me how that same person would perform as a part-time player or a minor leaguer. For the depth charts that go live in every article, I use the FanGraphs Depth Charts to determine the playing time for individual players. Since we’re talking about team construction, I can’t leave ZiPS to its own devices for an application like this. It’s the same reason I use modified depth charts for team projections in-season. There’s a probabilistic element in the ZiPS depth charts: Sometimes Joe Schmo will play a full season, sometimes he’ll miss playing time and Buck Schmuck will have to step in. But the basic concept is very straightforward.
What’s new in 2025? Outside of the myriad calibration updates, a lot of the additions were invisible to the public — quality of life things that allow me to batch run the projections faster and with more flexibility on the inputs. One consequence of this is that I will, for the first time ever, be able to do a preseason update that reflects spring training performance. It doesn’t mean a ton, but it means a little bit, and it’s something that Dan Rosenheck of The Economist demonstrated about a decade ago. Now that I can do a whole batch run of ZiPS on two computers in less than 36 hours, I can turn these around and get them up on FanGraphs within a reasonable amount of time, making it a feasible task. A tiny improvement is better than none!
The other change is that, starting with any projections that run in spring training, relievers will have save projections in ZiPS. One thing I’ve spent time doing is constructing a machine learning approach to saves, which focuses on previous roles, contract information, time spent with the team, and other pitchers available on the roster. This has been on my to do list for a while and I’m happy that I was able to get to it. It’s just impractical to do with these offseason team rundowns because the rosters will be in flux for the next four months.
Have any questions, suggestions, or concerns about ZiPS? I’ll try to reply to as many as I can reasonably address in the comments below. If the projections have been valuable to you now or in the past, I would also urge you to consider becoming a FanGraphs Member, should you have the ability to do so. It’s with your continued and much appreciated support that I have been able to keep so much of this work available to the public for so many years for free. Improving and maintaining ZiPS is a time-intensive endeavor and reader support allows me the flexibility to put an obscene number of hours into its development. It’s hard to believe I’ve been developing ZiPS for nearly half my life now! Hopefully, the projections and the things we’ve learned about baseball have provided you with a return on your investment, or at least a small measure of entertainment, whether it’s from being delighted or enraged.