Pitcher zStats Entering the Homestretch, Part 2 (The Stats!)
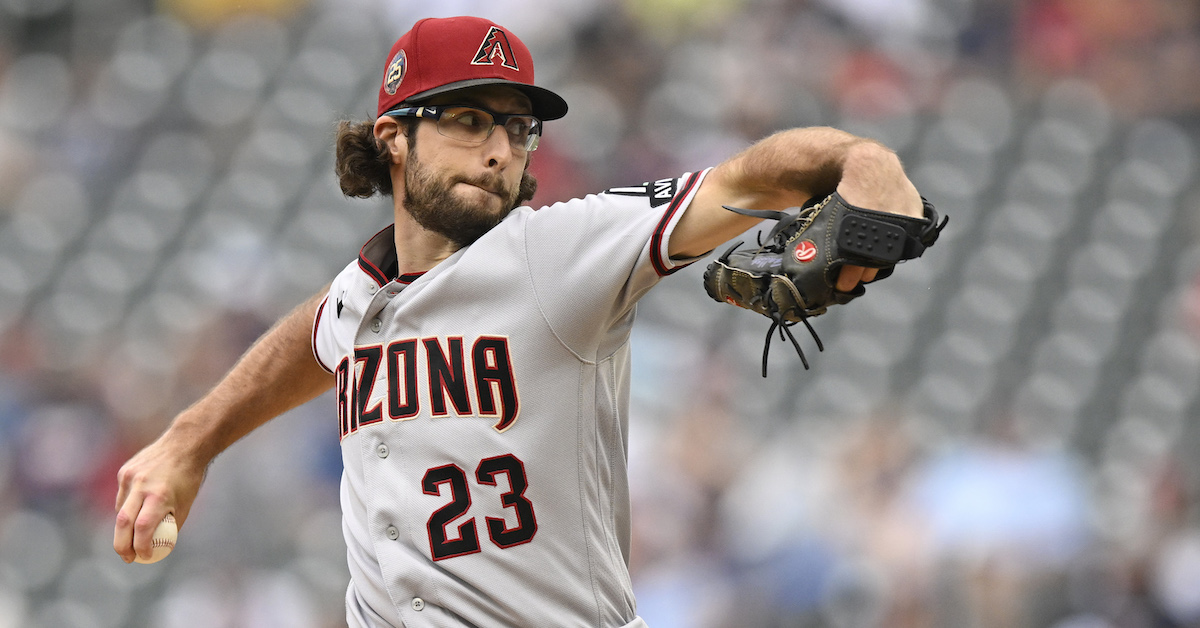
One of the strange things about projecting baseball players is that even results themselves are small samples. Full seasons result in specific numbers that have minimal predictive value, such as BABIP for pitchers. The predictive value isn’t literally zero; individual seasons form much of the basis of projections, whether math-y ones like ZiPS or simply our personal opinions on how good a player is. But we have to develop tools that improve our ability to explain some of these stats. It’s not enough to know that the number of home runs allowed by a pitcher is volatile; we need to know how and why pitchers allow homers beyond a general sense of pitching poorly or being Jordan Lyles.
Data like that which StatCast provides gives us the ability to get at what’s more elemental, such as exit velocities and launch angles and the like — things that are in themselves more predictive than their end products (the number of homers). StatCast has its own implementation of this kind of exercise in its various “x” stats. ZiPS uses slightly different models with a similar purpose, which I’ve dubbed zStats. (I’m going to make you guess what the z stands for!) The differences in the models can be significant. For example, when talking about grounders, balls hit directly toward the second base bag became singles 48.7% of the time from 2012 to ’19, with 51.0% outs and 0.2% doubles. But grounders hit 16 degrees to the “left” of the bag only became hits 10.6% of the time over the same stretch, and toward the second base side, it was 9.8%. ZiPS uses data like sprint speed when calculating hitter BABIP, because how fast a player is has an effect on BABIP and extra-base hits.
And why is this important and not just number-spinning? Knowing that changes in walk rates, home run rates, and strikeout rates stabilized far quicker than other stats was an important step forward in player valuation. That’s something that’s useful whether you work for a front office, are a hardcore fan, want to make some fantasy league moves, or even just a regular fan who is rooting for your faves. If we improve our knowledge of the basic molecular structure of a walk or a strikeout, then we can find players who are improving or struggling even more quickly, and provide better answers on why a walk rate or a strikeout rate has changed. This is useful data for me in particular because I obviously do a lot of work with projections, but I’m hoping this type of information is interesting to readers beyond that.
Yesterday, I went over how pitchers zStats for the first two months of the season performed over the last two months. Today, we’ll look at the updated data, through the games on August 10. Read the rest of this entry »